Enhancing Fault Isolation and Detection for Electric Powertrains of UAVs
information technology and software
Enhancing Fault Isolation and Detection for Electric Powertrains of UAVs (TOP2-305)
Embedding Failure Mode and Effect Analysis (FMEA) and Bayesian Framework for Real-time diagnostics/ prognostics
Overview
NASAs System-Wide Safety (SWS) project is developing innovative data solutions to assure safe, rapid, and repeatable access to a transformed National Airspace System. The increasing number of electric propulsion systems that will enter the airspace will require systems that ensure high safety standards in the low-altitude airspace. One element that can help ensure safety is proper diagnosis of failures via Fault Detection and Isolation (FDI). NASA Ames has developed a fault isolation approach for electric powertrains of unmanned aerial vehicles. It leverages a combination of Failure Mode and Effect Analysis (FMEA) and Bayesian Networks (BN) to create a dependability structure within a diagnostic framework-mapping fault and failure events from the FMEA within a BN. This framework helps the fault isolation process by identifying the probability of occurrence of specific fault or root causes given evidence observed through sensor signals.
The Technology
The tool developed through this work merges information from the electric propulsion system design phase with diagnostic tools. Information from the failure mode and effect analysis (FMEA) from the system design phase is embedded within a Bayesian network (BN). Each node in the network can represent either a fault, failure mode, root cause or effect, and the causal relationships between different elements are described through the connecting edges.
This novel approach can help Fault Detection and Isolation (FDI), producing a framework capable of isolating the cause of sub-system level fault and degradation.
This system:
Identifies and quantifies the effects of the identified hazards, the severity and probability of their effects, their root cause, and the likelihood of each cause;
Uses a Bayesian framework for fault detection and isolation (FDI);
Based on the FDI output, estimates health of the faulty component and predicts the remaining useful life (RUL) by also performing uncertainty quantification (UQ);
Identifies potential electric powertrain hazards and performs a functional hazard analysis (FHA) for unmanned aerial vehicles (UAVs)/Urban Air Mobility (UAM) vehicles.
Despite being developed for and demonstrated with an application to an electric UAV, the methodology is generalized and can be implemented in other domains, ranging from manufacturing facilities to various autonomous vehicles.
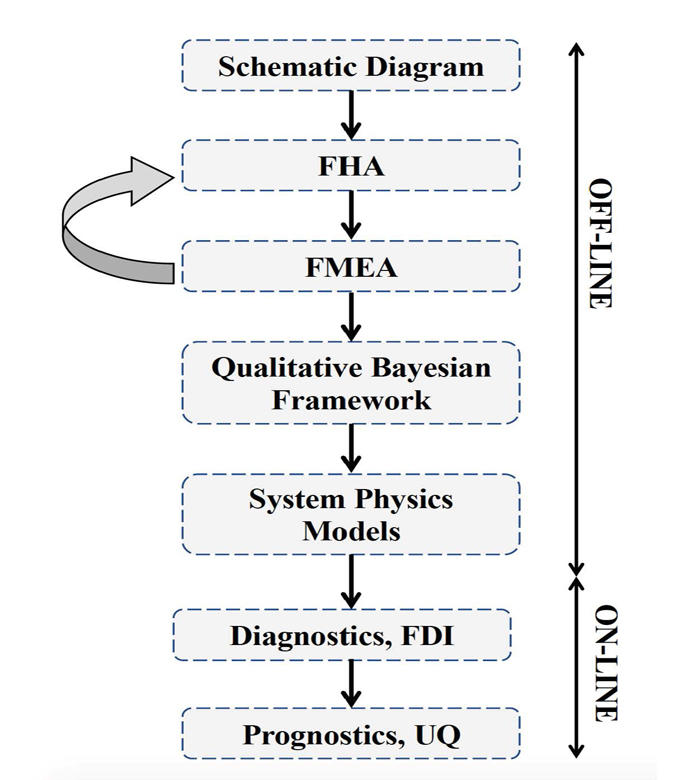
Benefits
- Method for rapidly understanding, isolating, and informing response to faults in a complex system
- Scalable to a larger system and the size is co-related to the schematic interactions between the different nodes
- Real-time diagnostics/ prognostics feedback that increases safety, decreases machine downtime, and improves profitability of a system
- Incorporates the age, usage rate, and specifications for components into decision making. The failure probabilities are updated based on these factors over the life of the component/ sub-system
- Can be adapted to a variety of components (including batteries, speed controllers, etc.) to provide value. The system is not overly constrained by a finite set of compliant hardware
- Generalizable to a broad spectrum of industry domains and use cases
- Enables timely observation of faults in mission critical scenarios (e.g., determining if UAV must land immediately or may proceed to destination)
- Automates the identification and pinpointing of faults in systems saving labor hours and enabling faster understanding of and reaction to faults
Applications
- Fault Diagnostics and Prognostics for Vehicle Systems Health Management
- Industrial Internet of Things (IIoTs)
- Aviation - Unmanned Aerial Vehicles (UAVs)
- Drone/Unmanned Aircraft Management (UAM) industry
- Automotive industry
- Commercial R&D for prognostic health management /condition-based maintenance
- Companies developing software products for system health management
- Aviation ground support
- Space industry (satellites)
- Manufacturing and factory settings (e.g., CNC machine monitoring), power plants, gas turbines, drilling system, and wind turbines
Technology Details
information technology and software
TOP2-305
ARC-18637-1
Chetan S. Kulkarni, Matteo Corbetta, Elinirina I. Robinson. Enhancing Fault Isolation for Health Monitoring of Electric Aircraft Propulsion by Embedding Failure Mode and Effect Analysis into Bayesian Networks. Proceedings of the Annual Conference of the Prognostics and Health Management Society, 12(1), Virtual, 2020.
Chetan S. Kulkarni, Matteo Corbetta, Elinirina I. Robinson. System Health Monitoring: Integrating FMEA into Bayesian Networks. IEEE Aerospace Conference, Virtual, 2021.
Chetan S. Kulkarni, Matteo Corbetta, Elinirina I. Robinson. System Health Monitoring: Integrating FMEA into Bayesian Networks. IEEE Aerospace Conference, Virtual, 2021.
Tags:
|
Similar Results

Reliable Geo-Limitation Algorithm for Unmanned Aircraft
Safeguard is an independent avionics equipment that can be easily ported to virtually any UA. The current prototype weighs approximately 1 lb (without hardware optimization). The invention innovations include formally verified algorithms to monitor and predict impending boundary violations through flight termination trajectory estimation. A system could be configured without sole reliance on the GPS to avoid known problems with GPS inaccuracies and unavailability. It can operate independent of the UA and any on-board components, such as the autopilot, for physical and logical separation from non-aviation-grade systems. The perimeter boundaries are described using polygons, which can approximate almost any shape, and there are practically no limits to the number of shapes and boundaries. The algorithms for establishing the validity of a boundary and for detecting proximity to all defined boundaries are based on rigorous mathematical models that have been formally verified.
Software required to operate cannot be licensed from NASA, the licensee must create and/or procure separately.

Safe2Ditch Technology
Safe2Ditch is a crash management system that resides on a small processor onboard a small Unmanned Aerial Vehicle (UAV). The system's exclusive mission is emergency management to get the vehicle safely to the ground in the event of an unexpected critical flight issue. It uses the remaining control authority and battery life of the crippled vehicle in an optimal way to reach the safest ditch location possible. It performs this mission autonomously, without any assistance from a safety pilot or ground station. In the event of an imminent crash, Safe2Ditch uses its intelligent algorithms, knowledge of the local area, and knowledge of the disabled vehicle's remaining control authority to select and steer to a crash location that minimizes risk to people and property. As it approaches the site, it uses machine vision to inspect the selected site to ensure that it is clear as expected.

Mitigating Risk in Commercial Aviation Operations
NASA’s newly developed software leverages flight operations data (e.g., SWIM Terminal Data Distribution System (STDDS) information), and with it, can predict aviation related risks, such as unstable approaches of flights. To do this, the software inputs the complex, multi-source STDDS data, and outputs novel prediction and outcome information.
The software converts the relatively inaccessible SWIM data from its native format that is not data science friendly into a format easily readable by most programs. The converted, model friendly data are then input into machine learning algorithms to enable risk prediction capabilities. The backend software sends the machine learning algorithm results to the front end software to display the results in appropriate user interfaces. These user interfaces can be deployed on different platforms including mobile phones and desktop computers and efficiently update models based on changes in the data.
To allow for visualization, the software uses a commercially available mapping API. The data are visualized in several different ways, including a heat map layer that shows the risk score, with higher risk in areas of higher flight density, a polyline layer, which shows flight paths, and markers that can indicate a flight’s location in real time, among other things. The related patent is now available to license. Please note that NASA does not manufacturer products itself for commercial sale.

Automata Learning in Generation of Scenario-Based Requirements in System Development
In addition, the higher the level of abstraction that developers can work from, as is afforded through the use of scenarios to describe system behavior, the less likely that a mismatch will occur between requirements and implementation and the more likely that the system can be validated. Working from a higher level of abstraction also provides that errors in the system are more easily caught, since developers can more easily see the big picture of the system.
This technology is a technique for fully tractable code generation from requirements, which has an application in other areas such as generation and verification of scripts and procedures, generation and verification of policies for autonomic systems, and may have future applications in the areas of security and software safety. The approach accepts requirements expressed as a set of scenarios and converts them to a process based description. The more complete the set of scenarios, the better the quality of the process based description that is generated. The proposed technology using automata learning to generate possible additional scenarios can be useful in completing the description of the requirements.

Improved Ground Collision Avoidance System
This critical safety tool can be used for a wider variety of aircraft, including general aviation, helicopters, and unmanned aerial vehicles (UAVs) while also improving performance in the fighter aircraft currently using this type of system.
Demonstrations/Testing
This improved approach to ground collision avoidance has been demonstrated on both small UAVs and a Cirrus SR22 while running the technology on a mobile device. These tests were performed to the prove feasibility of the app-based implementation of this technology. The testing also characterized the flight dynamics of the avoidance maneuvers for each platform, evaluated collision avoidance protection, and analyzed nuisance potential (i.e., the tendency to issue false warnings when the pilot does not consider ground impact to be imminent).
Armstrong's Work Toward an Automated Collision Avoidance System
Controlled flight into terrain (CFIT) remains a leading cause of fatalities in aviation, resulting in roughly 100 deaths each year in the United States alone. Although warning systems have virtually eliminated CFIT for large commercial air carriers, the problem still remains for fighter aircraft, helicopters, and GAA.
Innovations developed at NASAs Armstrong Flight Research Center are laying the foundation for a collision avoidance system that would automatically take control of an aircraft that is in danger of crashing into the ground and fly it—and the people inside—to safety. The technology relies on a navigation system to position the aircraft over a digital terrain elevation data base, algorithms to determine the potential and imminence of a collision, and an autopilot to avoid the potential collision. The system is designed not only to provide nuisance-free warnings to the pilot but also to take over when a pilot is disoriented or unable to control the aircraft.
The payoff from implementing the system, designed to operate with minimal modifications on a variety of aircraft, including military jets, UAVs, and GAA, could be billions of dollars and hundreds of lives and aircraft saved. Furthermore, the technology has the potential to be applied beyond aviation and could be adapted for use in any vehicle that has to avoid a collision threat, including aerospace satellites, automobiles, scientific research vehicles, and marine charting systems.