Search
instrumentation
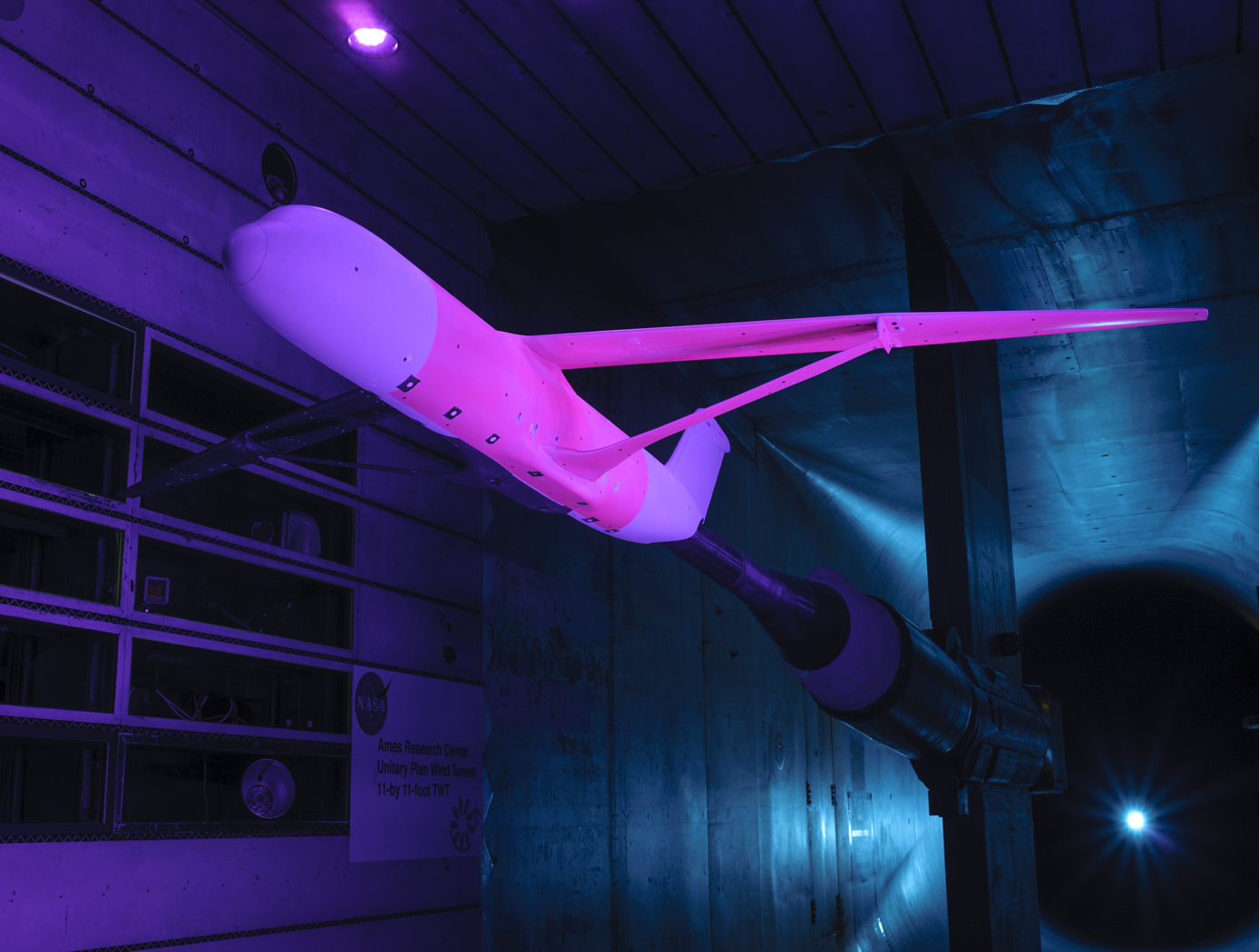
Calculation of Unsteady Aerodynamic Loads Using Fast-Response Pressure-Sensitive Paint (PSP)
Traditionally, unsteady pressure transducers have been the instrumentation of choice for investigating unsteady flow phenomena which can be time-consuming and expensive. The ability to measure and compute these flows has been a long-term challenge for aerospace vehicle designers and manufacturers. Results using only the pressure transducers are prone to inaccuracies, providing overly conservative load predictions in some cases and underestimating load predictions in other areas depending on the flow characteristics. NASA Ames has developed a new state-of-the-art method for measuring fluctuating aerodynamic-induced pressures on wind tunnel models using unsteady Pressure Sensitive Paint (uPSP). The technology couples recent advances in high-speed cameras, high-powered energy sources, and fast response pressure-sensitive paint. The unsteady pressure-sensitive paint (uPSP) technique has emerged as a powerful tool to measure flow, enabling time-resolved measurements of unsteady pressure fluctuations within a dense grid of spatial points on a wind tunnel model. The invention includes details surrounding uPSP processing. This technique enables time-resolved measurements of unsteady pressure fluctuations within a dense grid of spatial points representing the wind tunnel model. Since uPSP is applied by a spray gun, it is continuously distributed. With this approach, if the model geometry can be painted, viewed from a camera, and excited by a lamp source, uPSP data can be collected. Unsteady PSP (uPSP) has the ability to determine more accurate integrated unsteady loads.
aerospace
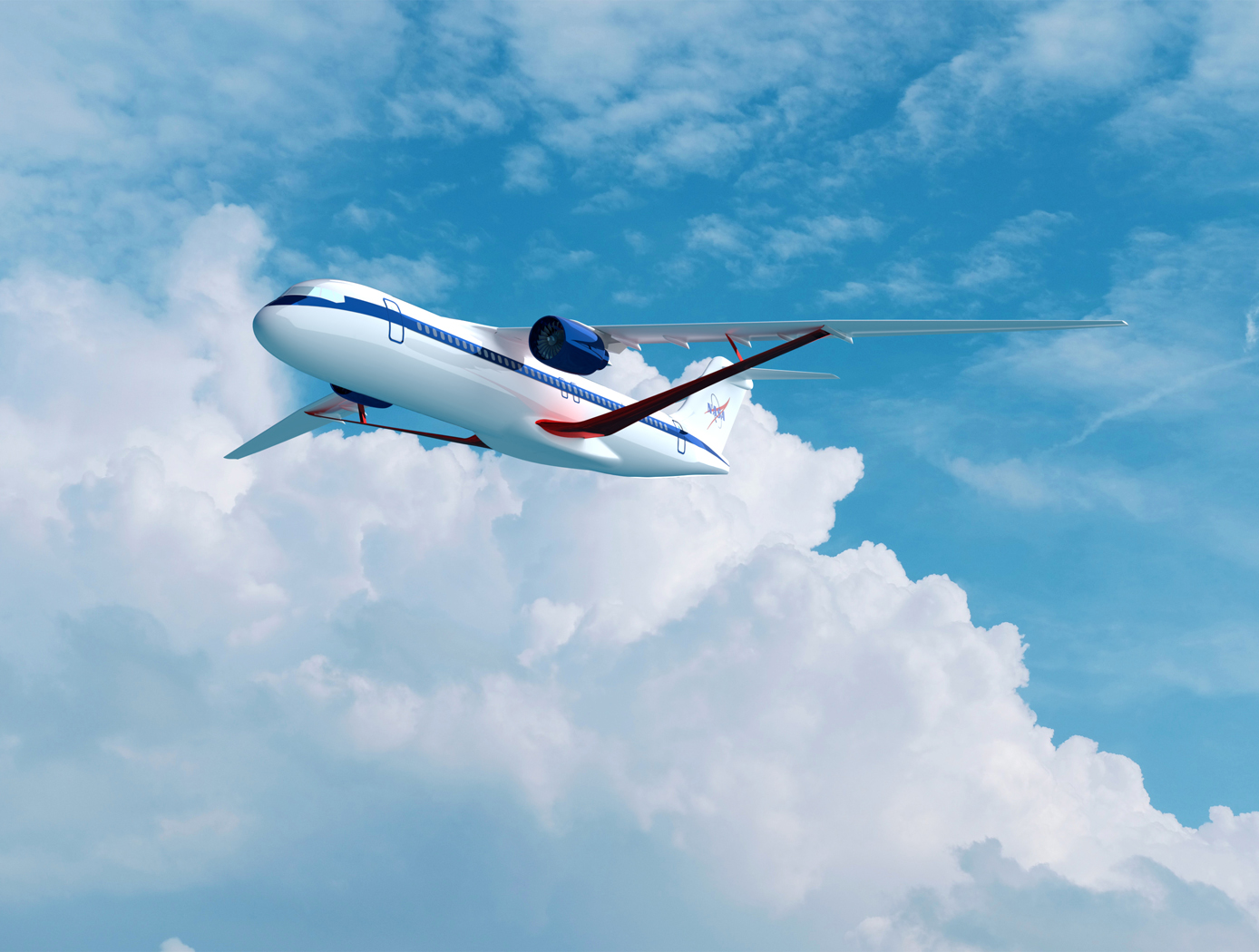
Methods for Predicting Transonic Flutter Using Simple Data Models
Transonic flutter is a pacing item in transport aircraft design in that it is crucial to characterize this phenomenon for each aircraft to prevent catastrophic failure. Aerodynamic study of flows around airfoils is a canonical problem that entails both experimental and computational approaches. While the transonic flutter prediction can be more accurate with high-fidelity Computational Fluid Dynamics (CFD) methods than with unsteady potential flow methods, the computational cost is high. Therefore, computationally efficient methods for transonic flutter prediction continue to be of high interest to the aircraft design community. NASA Ames has developed a novel method that eliminates the need for expensive calculations of aerodynamics of wing flutter, which typically takes tens of hours on a supercomputer. Such calculations are now replaced by machine-learning-based closed form solutions that provide the solution almost instantaneously. The technology presents a new approach to predict the flow around pitching NACA00 series airfoils. NACA airfoils are generally symmetric, and thus they do not possess camber. However, the invention can readily extend to wings with camber. This novel data modeling approach is orders of magnitude faster than the traditional CFD approach of predicting aerodynamic effects of transonic pitching airfoils. The data model is based on a subset of unsteady CFD simulations that train the model. The trained model then resolves the pitching airfoil in time for any other set on the order of a second, as compared with a complete CFD simulation that typically takes 30 hours on a supercomputer. The data model is demonstrated in this invention for transonic flow corresponding to Mach number of 0.755 over pitching NACA00 series airfoils for a reduced frequency range typical of flutter, i.e., k lies in the range 0.02 - 0.25.