Computer Vision Lends Precision to Robotic Grappling
information technology and software
Computer Vision Lends Precision to Robotic Grappling (MSC-TOPS-114)
AI analysis of live camera feed yields delta commands to human operator
Overview
Innovators at NASA Johnson Space Center (JSC) have developed computer vision software that derives target posture determinations quickly and then instructs an operator how to properly align a robotic end-effector with a target that they are trying to grapple. As an added benefit, the softwares object identification capability can also help detect physical defects on targets.
This technology was originally created to aid robotic arm operators aboard the International Space Station (ISS) that relied more heavily upon grappling instructional maneuvers derived from flight controllers on the ground at JSCs Mission Control Center (MCC). Despite the aid of computer-based models to predict the alignment of both robotic arm and target, iterative realignment procedures were often required to correct botched grapple operations, costing valuable time.
To solve this problem, NASAs computer vision software analyzes the live camera feed from the robotic arms single borescope camera and provides the operator with the delta commands required for an ideal grasp operation. This process is aided by a machine learning component that monitors the camera feed for any of the ISSs potential target fixtures. Once a target fixture is identified, proper camera and target parameters are automatically sequenced to prepare for grasping operations.
The Technology
The goal of this computer vision software is to take the guesswork out of grapple operations aboard the ISS by providing a robotic arm operator with real-time pose estimation of the grapple fixtures relative to the robotic arms end effectors. To solve this Perspective-n-Point challenge, the software uses computer vision algorithms to determine alignment solutions between the position of the camera eyepoint with the position of the end effector as the borescope camera sensors are typically located several centimeters from their respective end effector grasping mechanisms.
The software includes a machine learning component that uses a trained regional Convolutional Neural Network (r-CNN) to provide the capability to analyze a live camera feed to determine ISS fixture targets a robotic arm operator can interact with on orbit. This feature is intended to increase the grappling operational range of ISSs main robotic arm from a previous maximum of 0.5 meters for certain target types, to greater than 1.5 meters, while significantly reducing computation times for grasping operations.
Industrial automation and robotics applications that rely on computer vision solutions may find value in this softwares capabilities. A wide range of emerging terrestrial robotic applications, outside of controlled environments, may also find value in the dynamic object recognition and state determination capabilities of this technology as successfully demonstrated by NASA on-orbit.
This computer vision software is at a technology readiness level (TRL) 6, (system/sub-system model or prototype demonstration in an operational environment.), and the software is now available to license. Please note that NASA does not manufacture products itself for commercial sale.
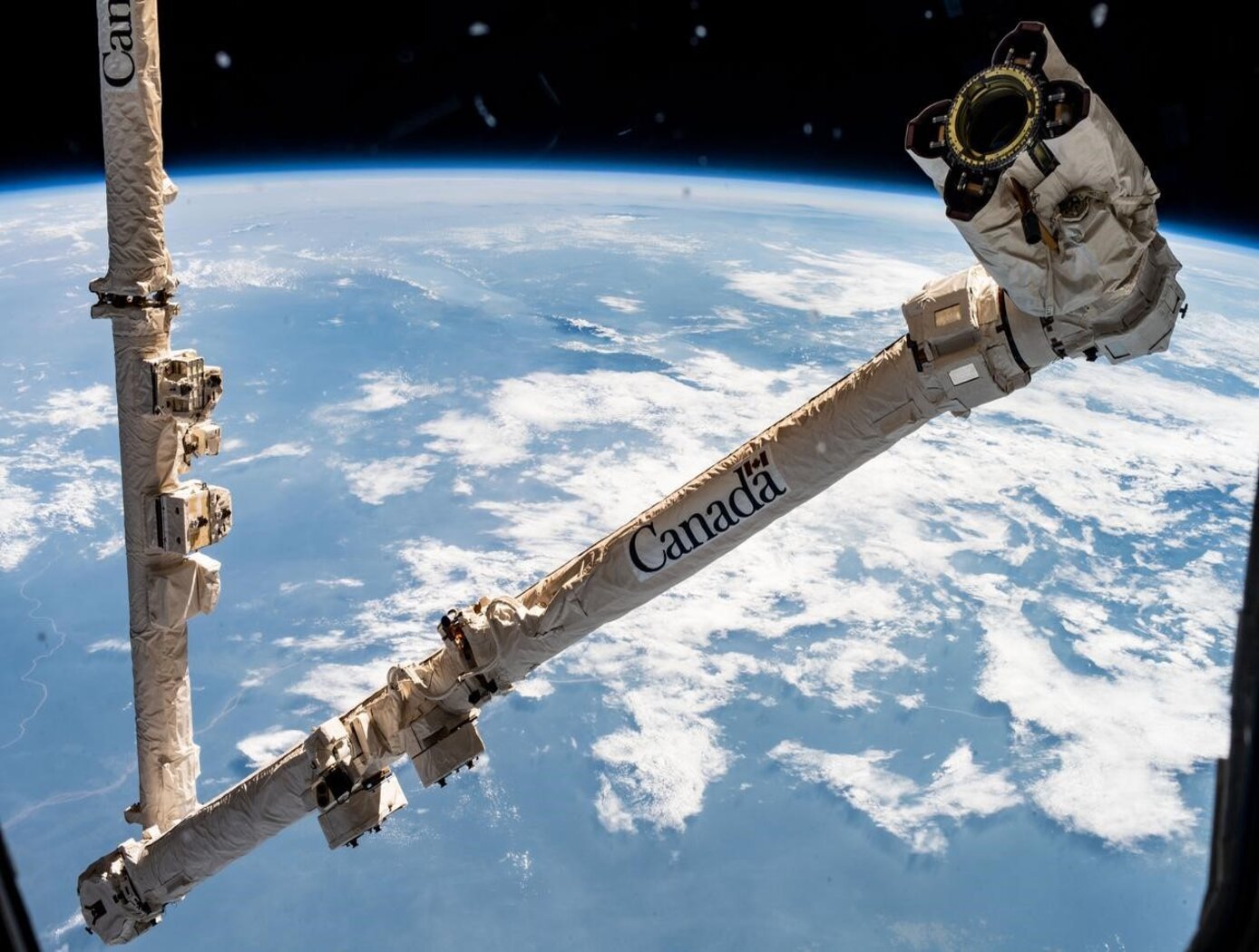
![Shown: A target is acquired by the vision system. The parameters [X, Y, Z] (cm) are calculated and delta commands are fed to the human operator to move the robotic arm end-effector to grapple a moving target. Shown: A target is acquired by the vision system. The parameters [X, Y, Z] (cm) are calculated and delta commands are fed to the human operator to move the robotic arm end-effector to grapple a moving target.](https://technology.nasa.gov/t2media/tops/img/MSC-TOPS-114/bck.jpg)
Benefits
- Machine-guided efficiency: high fidelity position data allows human flight controllers to eliminate errors and save mission time.
- Trainable object recognition: a neural network capable of learning new targets automatically identifies objects as they enter a cameras field of view.
- Real-time tracking: recognized objects can be tracked, and positional data updated from frame to frame.
- Dynamic feedback: flight controllers are shown solution quality of target object position calculations.
Applications
- Orbital service: grasping operations for deployment or capture of spacecraft, satellites, and debris; robotic in-situ assembly
- Hazardous environments: robotic vision systems for mining, power generation, marine, reconnaissance and military
- Vehicle docking: mechanized capture, transport, and storage solutions
- Industrial automation: robotic assembly and manufacturing, inspection, automated local area transport
- Telesurgery: robotic vision systems to assist surgeons remotely in the operative field
Technology Details
information technology and software
MSC-TOPS-114
MSC-27184-1
Lucier et. al. International Space Station (ISS) Robotics Development Operations Team Results in Robotic Remote Sensing, Control, and Semi-Automated Ground Control Techniques. 16th International Conference on Space Operations, Cape Town, South Africa. May 3-5, 2021.