Vision-based Approach and Landing System (VALS)
Aerospace
Vision-based Approach and Landing System (VALS) (TOP2-322)
Alternative Position, Navigation, and Timing (APNT) solution for Advanced Air Mobility aircraft in environments where GPS is not available
Overview
There are certain situations when landing an Advanced Air Mobility (AAM) aircraft is required to be performed without assistance from GPS data. For example, AAM aircraft flying in an urban environment with tall buildings and narrow canyons may affect the ability of the AAM aircraft to effectively use GPS to access a landing area. Incorporating a vision-based navigation method, NASA Ames has developed a novel Alternative Position, Navigation, and Timing (APNT) solution for AAM aircraft in environments where GPS is not available. The technology employs an aircraft-mounted camera to visually detect known features in the landing area, using a computer vision method called Coplanar Pose from Orthography and Scaling with Iterations (COPOSIT) to determine the position and orientation of the aircraft relative to the features detected from the camera images, and an extended Kalman filter to predict and correct the estimated position, velocity, and orientation of the aircraft.
The Technology
The novel Vision-based Approach and Landing System (VALS) provides Advanced Air Mobility (AAM) aircraft with an Alternative Position, Navigation, and Timing (APNT) solution for approach and landing without relying on GPS. VALS operates on multiple images obtained by the aircraft’s video camera as the aircraft performs its descent. In this system, a feature detection technique such as Hough circles and Harris corner detection is used to detect which portions of the image may have landmark features. These image areas are compared with a stored list of known landmarks to determine which features correspond to the known landmarks. The world coordinates of the best matched image landmarks are inputted into a Coplanar Pose from Orthography and Scaling with Iterations (COPOSIT) module to estimate the camera position relative to the landmark points, which yields an estimate of the position and orientation of the aircraft. The estimated aircraft position and orientation are fed into an extended Kalman filter to further refine the estimation of aircraft position, velocity, and orientation. Thus, the aircraft’s position, velocity, and orientation are determined without the use of GPS data or signals. Future work includes feeding the vision-based navigation data into the aircraft’s flight control system to facilitate aircraft landing.
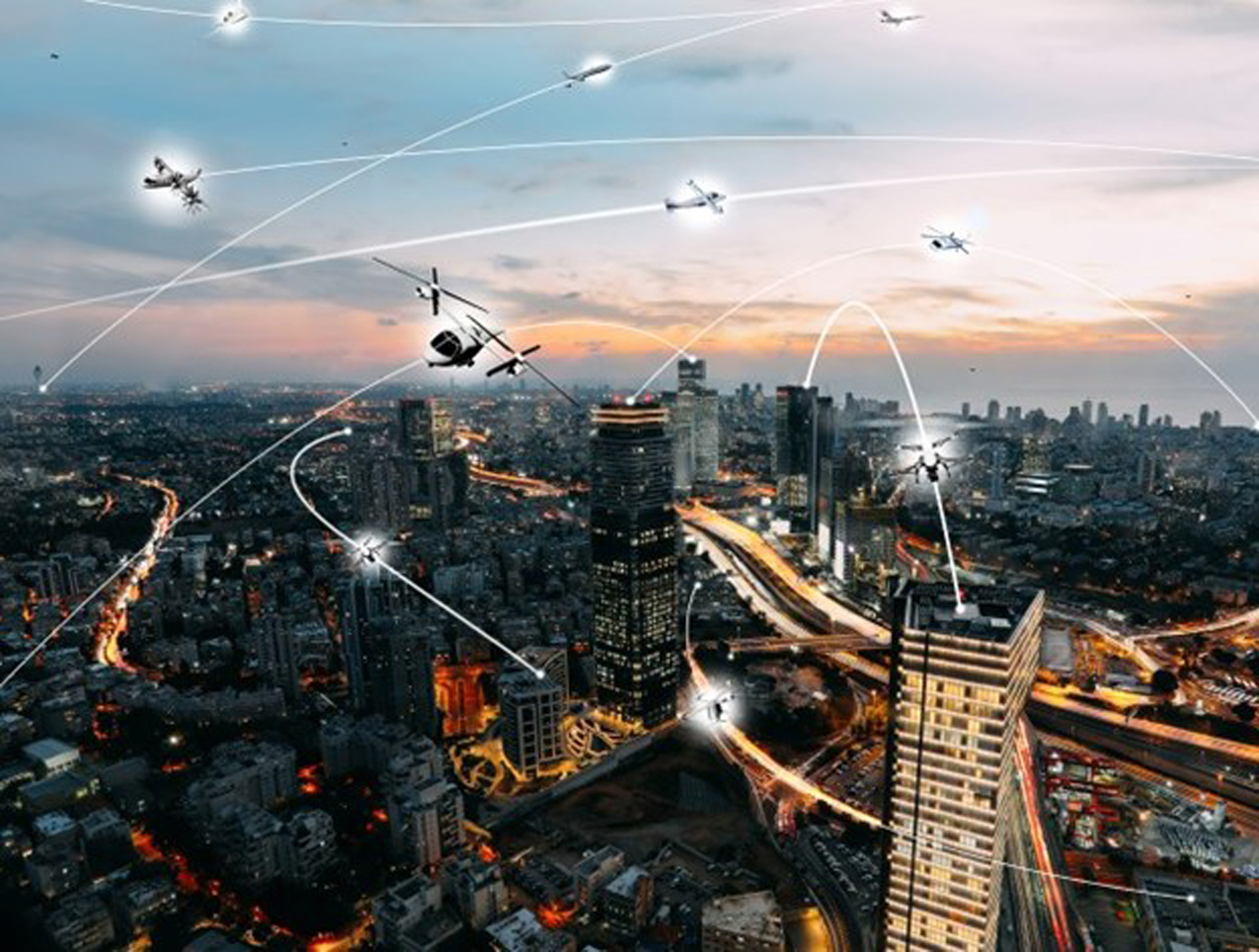
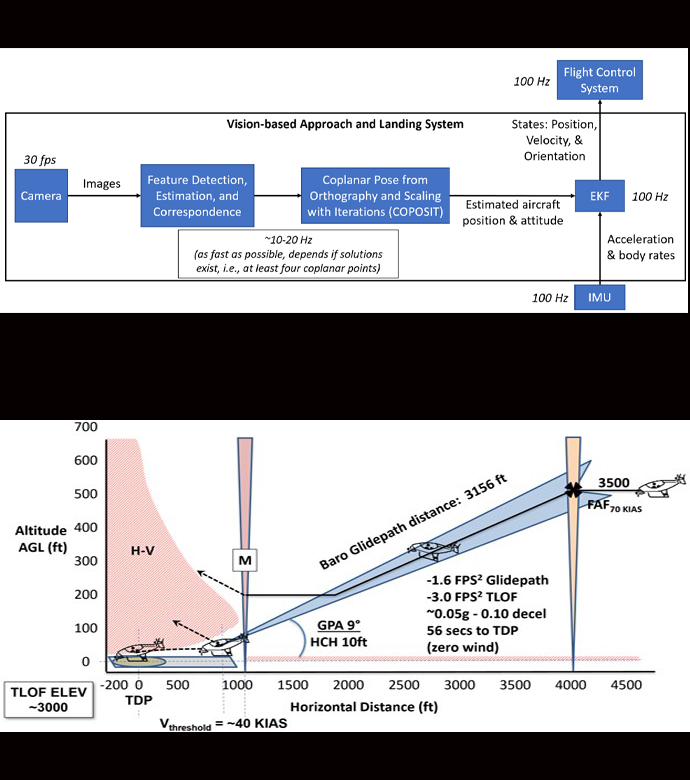
Benefits
- Precision landing solution in GPS-denied or complex environments: replacement or backup to GPS-based or ground-based visual navigation in automated approach and landing systems using onboard vision and sensors
- Enhanced safety: provides AAM aircraft with an Alternative Position, Navigation, and Timing (APNT) solution for approach and landing without relying on GPS
- Precise position, velocity, and orientation of the aircraft is determined without access to GPS compared to conventional systems
- Near real-time operation: applies the computer vision method Coplanar Pose from Orthography and Scaling with Iterations (COPOSIT) with feature detection methods
- Combining IMU (inertial measurement unit) with vision creates a sensor fusion navigation solution for GPS-denied environments
Applications
- Advanced Air Mobility (AAM)
- Urban Air Mobility (UAM) aircraft (e.g., drones and other unmanned aerial vehicles (UAVs), and electric vertical takeoff and landing (eVTOL) aircraft)
- Air-Taxi industry
- Commercial aircraft with downward-facing cameras may also apply this approach and incorporate a landing system based on the landing lights or fiducials on the runway
- Airports, heliports, and vertiports with landmarks or landing lights or fiducials can leverage the technology to assist incoming aircraft during approach and landing
Technology Details
Aerospace
TOP2-322
ARC-18891-1
Simulated Vision-based Approach and Landing System for Advanced Air Mobility
https://arc.aiaa.org/doi/abs/10.2514/6.2023-2195
https://ntrs.nasa.gov/api/citations/20220017713/downloads/main%202023%20AIAA%20DS%20r10ek.pdf
https://ntrs.nasa.gov/api/citations/20220018454/downloads/2023%20AIAA%20Scitech%20VALS%20Presentation%20r5ek.pdf
VSLAM and Vision-based Approach and Landing for Advanced Air Mobility
https://arc.aiaa.org/doi/abs/10.2514/6.2023-2196
https://ntrs.nasa.gov/api/citations/20220017714/downloads/main%20VSLAM%20r12ek.pdf
https://ntrs.nasa.gov/api/citations/20220018455/downloads/2023%20AIAA%20Scitech%20VSLAM%20VAL%20Presentation%20r5ek.pdf
Vision-Based Precision Approach and Landing for Advanced Air Mobility
https://arc.aiaa.org/doi/abs/10.2514/6.2022-0497
https://ntrs.nasa.gov/api/citations/20210025114/downloads/main%20r13_compressed.pdf
https://ntrs.nasa.gov/api/citations/20210025280/downloads/2022%20AIAA%20Scitech%20Presentation%20r3ek.pdf
https://arc.aiaa.org/doi/abs/10.2514/6.2023-2195
https://ntrs.nasa.gov/api/citations/20220017713/downloads/main%202023%20AIAA%20DS%20r10ek.pdf
https://ntrs.nasa.gov/api/citations/20220018454/downloads/2023%20AIAA%20Scitech%20VALS%20Presentation%20r5ek.pdf
VSLAM and Vision-based Approach and Landing for Advanced Air Mobility
https://arc.aiaa.org/doi/abs/10.2514/6.2023-2196
https://ntrs.nasa.gov/api/citations/20220017714/downloads/main%20VSLAM%20r12ek.pdf
https://ntrs.nasa.gov/api/citations/20220018455/downloads/2023%20AIAA%20Scitech%20VSLAM%20VAL%20Presentation%20r5ek.pdf
Vision-Based Precision Approach and Landing for Advanced Air Mobility
https://arc.aiaa.org/doi/abs/10.2514/6.2022-0497
https://ntrs.nasa.gov/api/citations/20210025114/downloads/main%20r13_compressed.pdf
https://ntrs.nasa.gov/api/citations/20210025280/downloads/2022%20AIAA%20Scitech%20Presentation%20r3ek.pdf
Tags:
|
|
Related Links:
|