Airborne Machine Learning Estimates for Local Winds and Kinematics
Robotics Automation and Control
Airborne Machine Learning Estimates for Local Winds and Kinematics (TOP2-277)
GPS-free Estimations using COTS Sensors for UAS and Air Taxi Operations in Complex Urban Environments
Overview
Future Unmanned Aerial Systems (UAS) and air taxis will require advanced onboard autonomy to operate safely within complex and dynamic urban environments. Urban landscapes are dynamic and constantly evolving. In addition to multi-directional, intense, and seemingly unpredictable winds often created in urban canyons, an exact knowledge of current building sizes, shapes, and positions is also often unavailable for real-time navigation. NASA Ames has developed a novel system, MAESTRO: MAchine learning ESTimations for uRban Operations, which not only improves the flight safety of UAS and air taxis in complex dynamic environments but also allows them to make smart and rapid on-board estimations of the local surrounding winds and vehicle kinematics using commercial off-the-shelf (COTS) sensors and advanced onboard computing.
The Technology
The MAchine learning ESTimations for uRban Operations (MAESTRO) system is a novel approach that couples commodity sensors with advanced algorithms to provide real-time onboard local wind and kinematics estimations to a vehicle's guidance and navigation system. Sensors and computations are integrated in a novel way to predict local winds and promote safe operations in dynamic urban regions where Global Positioning System/Global Navigation Satellite System (GPS/GNSS) and other network communications may be unavailable or are difficult to obtain when surrounded by tall buildings due to multi-path reflections and signal diffusion. The system can be implemented onboard an Unmanned Aerial Systems (UAS) and once airborne, the system does not require communication with an external data source or the GPS/GNSS. Estimations of the local winds (speed and direction) are created using inputs from onboard sensors that scan the local building environment. This information can then be used by the onboard guidance and navigation system to determine safe and energy-efficient trajectories for operations in urban and suburban settings. The technology is robust to dynamic environments, input noise, missing data, and other uncertainties, and has been demonstrated successfully in lab experiments and computer simulations.
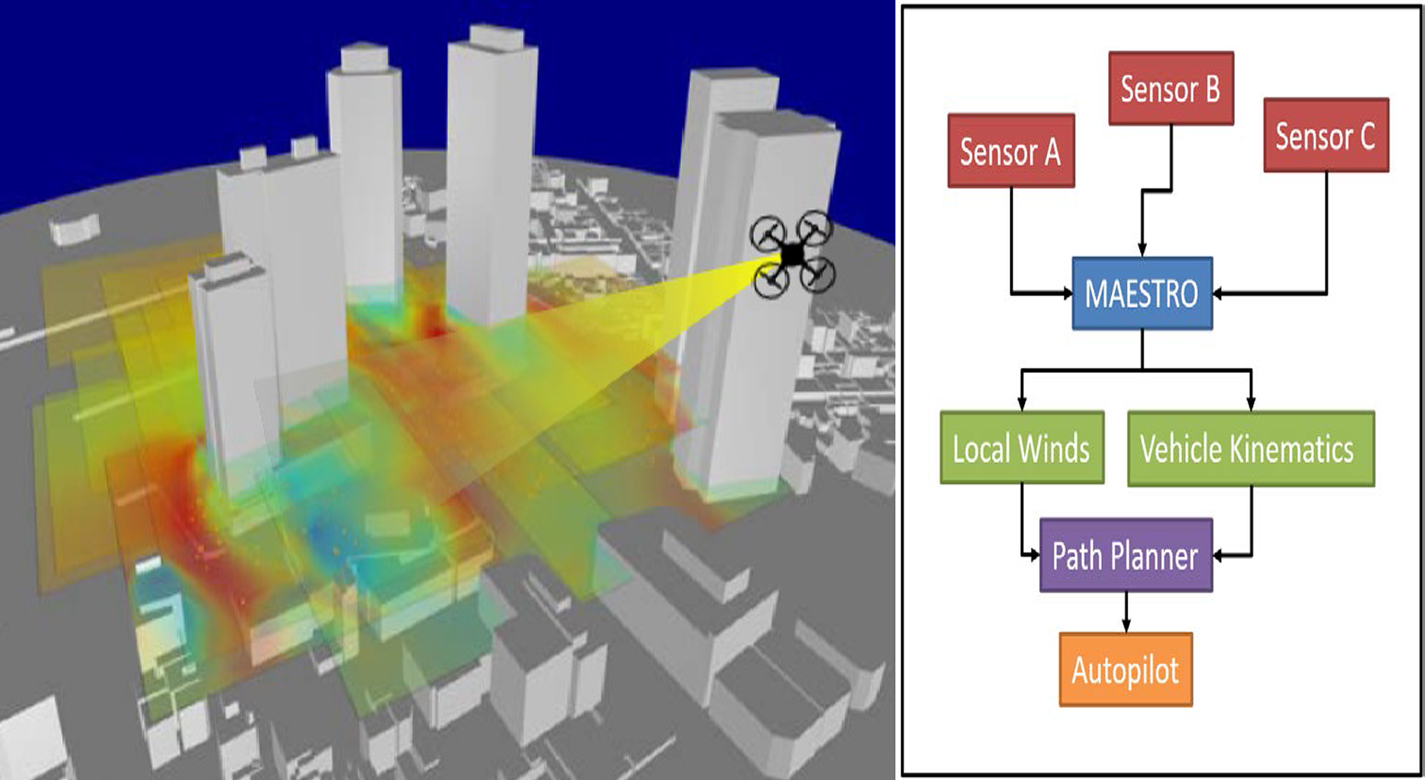
Benefits
- Accurate and fast estimates of the local wind environment and vehicle kinematics
- Uses advanced machine learning algorithms linked with commodity sensors
- Allows for safe navigation through and around complex urban environments
- Allows UAS and air taxis to estimate winds based on geometry of surroundings
- Provides all-azimuth predictions
- Integrates well with existing airborne sensors
- Requires no external communication or network after deployment
- Provides robust and efficient predictions for dynamic (or possibly unknown) urban geometry
- Runs efficiently (less than 0.1 sec) on commodity portable computer hardware
- Produces actionable advisories that fit seamlessly into the GNC process stream
- Provides GPS-free position and attitude estimations
Applications
- Urban air taxis / Urban Air Mobility (UAM)
- Urban UAS Package delivery
- UAS Emergency Medical Services (EMS) services (like: toxic plume/smoke/ash prediction for urban fires and pollution spills)
- UAS-based surveillance and infrastructure inspection services
- Defense and Intelligence operations
- Ship air wake predictions for safe maritime UAS operations
- Landing zone wind field predictions for precision parachute airdrops
- Detailed wind field predictions at urban airports
- Wind predictions in mountain valleys, canyons, etc.
- Improved local ballistic trajectory predictions
Technology Details
Robotics Automation and Control
TOP2-277
ARC-17836-1
Patent-pending
"Patent Only/No Software"
"Patent Only/No Software"
Tags:
|
Similar Results

Mitigating Risk in Commercial Aviation Operations
NASA’s newly developed software leverages flight operations data (e.g., SWIM Terminal Data Distribution System (STDDS) information), and with it, can predict aviation related risks, such as unstable approaches of flights. To do this, the software inputs the complex, multi-source STDDS data, and outputs novel prediction and outcome information.
The software converts the relatively inaccessible SWIM data from its native format that is not data science friendly into a format easily readable by most programs. The converted, model friendly data are then input into machine learning algorithms to enable risk prediction capabilities. The backend software sends the machine learning algorithm results to the front end software to display the results in appropriate user interfaces. These user interfaces can be deployed on different platforms including mobile phones and desktop computers and efficiently update models based on changes in the data.
To allow for visualization, the software uses a commercially available mapping API. The data are visualized in several different ways, including a heat map layer that shows the risk score, with higher risk in areas of higher flight density, a polyline layer, which shows flight paths, and markers that can indicate a flight’s location in real time, among other things. The related patent is now available to license. Please note that NASA does not manufacturer products itself for commercial sale.

Unmanned Aerial Systems (UAS) Traffic Management
NASA Ames has developed an Autonomous Situational Awareness Platform system for a UAS (ASAP-U), a traffic management system to incorporate Unmanned Aerial Systems (UASs) into the National Airspace System. The Autonomous Situational Awareness Platform (ASAP) is a system that combines existing navigation technology (both aviation and maritime) with new procedures to safely integrate Unmanned Aerial Systems (UASs) with other airspace vehicles. It uses a module called ASAP-U, which includes a transmitter, receivers, and various links to other UAS systems. The module collects global positioning system GPS coordinates and time from a satellite antenna, and this data is fed to the UAS's flight management system for navigation. The ASAP-U module autonomously and continuously sends UAS information via a radio frequency (RF) antenna using Self-Organized Time Division Multiple Access (SOTDMA) to prevent signal overlap. It also receives ASAP data from other aircraft. In case of transmission overload, priority is given to closer aircraft. Additionally, the module can receive weather data, navigational aid data, terrain data, and updates to the UAS flight plan. The collected data is relayed to the flight management system, which includes various databases and a navigation computer to calculate necessary flight plan modifications based on regulations, right-of-way rules, terrain, and geofencing. Conflicts are checked against databases, and if none are found, the flight plan is implemented. If conflicts arise, modifications can be made. The ASAP-U module continuously receives and transmits data, including UAS data and data from other aircraft, to detect conflicts with other aircraft, terrain, weather, and geofencing. Based on this information, the flight management system determines the need for course adjustments and the flight control system executes them for a safe flight route.

Vision-based Approach and Landing System (VALS)
The novel Vision-based Approach and Landing System (VALS) provides Advanced Air Mobility (AAM) aircraft with an Alternative Position, Navigation, and Timing (APNT) solution for approach and landing without relying on GPS. VALS operates on multiple images obtained by the aircraft’s video camera as the aircraft performs its descent. In this system, a feature detection technique such as Hough circles and Harris corner detection is used to detect which portions of the image may have landmark features. These image areas are compared with a stored list of known landmarks to determine which features correspond to the known landmarks. The world coordinates of the best matched image landmarks are inputted into a Coplanar Pose from Orthography and Scaling with Iterations (COPOSIT) module to estimate the camera position relative to the landmark points, which yields an estimate of the position and orientation of the aircraft. The estimated aircraft position and orientation are fed into an extended Kalman filter to further refine the estimation of aircraft position, velocity, and orientation. Thus, the aircraft’s position, velocity, and orientation are determined without the use of GPS data or signals. Future work includes feeding the vision-based navigation data into the aircraft’s flight control system to facilitate aircraft landing.

Adaptive wind estimation for small unmanned aerial systems using motion data
The technology presents an on-board estimation, navigation and control architecture for multi-rotor drones flying in an urban environment. It consists of adaptive algorithms to estimate the vehicle's aerodynamic drag coefficients with respect to still air and urban wind components along the flight trajectory, with guaranteed fast and reliable convergence to the true values. Navigation algorithms generate feasible trajectories between given way-points that take into account the estimated wind. Control algorithms track the generated trajectories as long as the vehicle retains a sufficient number of functioning rotors that are capable of compensating for the estimated wind. The technology provides a method of measuring wind profiles on a drone using existing motion sensors, like the inertial measurement unit (IMU), rate gyroscope, etc., that are observably necessary for any drone to operate. The algorithms are used to estimate wind around the drone. They can be used for stability or trajectory calculations, and are adaptable for use with any UAV regardless of the knowledge of weight and inertia. They further provide real-time calculations without additional sensors. The estimation method is implemented using onboard computing power. It rapidly converges to true values, is computationally inexpensive, and does not require any specific hardware or specific vehicle maneuvers for the convergence. All components of this on-board system are computationally effective and are intended for a real time implementation. The method's software is developed in a Matlab/Simulink environment, and has executable versions, which are suitable for majority of existing onboard controllers. The algorithms were tested in simulations.

Low Weight Flight Controller Design
Increasing demand for smaller UAVs (e.g., sometimes with wingspans on the order of six inches and weighing less than one pound) generated a need for much smaller flight and sensing equipment. NASA Langley's new sensing and flight control system for small UAVs includes both an active flight control board and an avionics sensor board. Together, these compare the status of the UAVs position, heading, and orientation with the pre-programmed data to determine and apply the flight control inputs needed to maintain the desired course.
To satisfy the small form-factor system requirements, micro-electro-mechanical systems (MEMS) are used to realize the various flight control sensing devices. MEMS-based devices are commercially available single-chip devices that lend themselves to easy integration onto a circuit board. The system uses less energy than current systems, allowing solar panels planted on the vehicle to generate the systems power. While the lightweight technology was designed for smaller UAVs, the sensors could be distributed throughout larger UAVs, depending on the application.