Algorithms for stabilizing intelligent networks
robotics automation and control
Algorithms for stabilizing intelligent networks (GSC-TOPS-6)
Biologically inspired algorithms for stabilizing intelligent, learning networks
Overview
Inspired by psychology, these algorithms could be developed and applied towards creating stable, predictable, and artificially intelligent networks. These algorithms collectively represent ways for intelligent systems to identify and correct unpredictable or unstable behaviors, creating stable emotional states that govern behaviors with given specific circumstances, and establishing an evolvable synthetic neural network that can eventually be scaled from low-level functions to higher level decision making processes. These algorithms could be key to research in autonomous spacecraft, nanorobotic swarms, and sensor networks.
The Technology
Some of the current challenges faced by research in artificial intelligence and autonomous control systems include providing self control, resilience, adaptability, and stability for intelligent systems, especially over a long period of time, in changing environments. The Evolvable Neural Software System (ENSS), Formulation for Emotion Embedding in Logic Systems (FEELS), Stability Algorithm for Neural Entities (SANE), and the Logic Expansion for Autonomously Reconfigurable Neural Systems (LEARNS) are foundations for tackling some of these challenges, by providing the basic algorithms evolvable systems could use to manage its own behavior.
These algorithms would allow networks to self regulate, noticing unusual behavior and the circumstances that may have caused that behavior, and then correcting to behave more predictably when similar circumstances are encountered. The process is similar to how psychology in organisms evolved iteratively, eventually finding and keeping better responses to given stimuli.
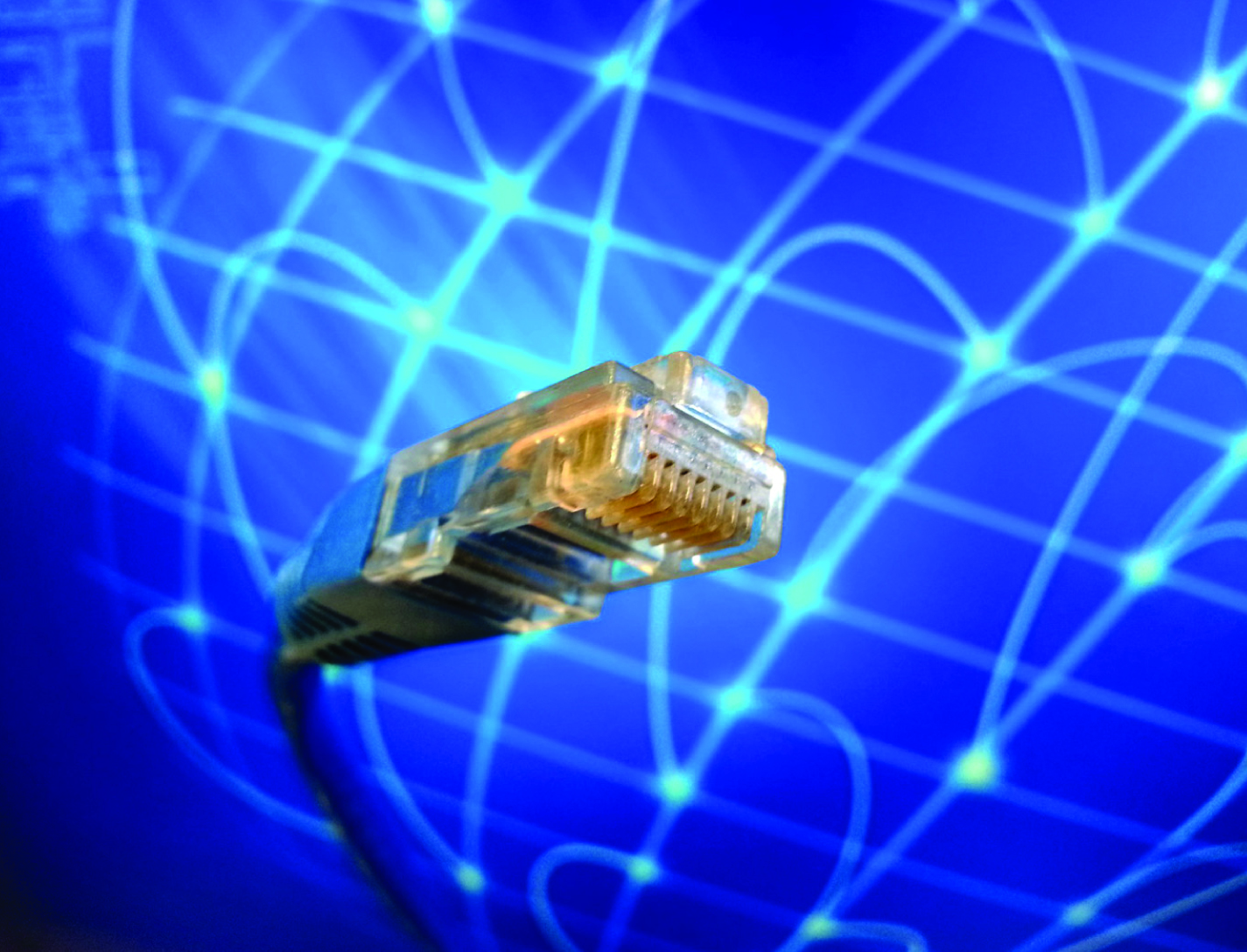
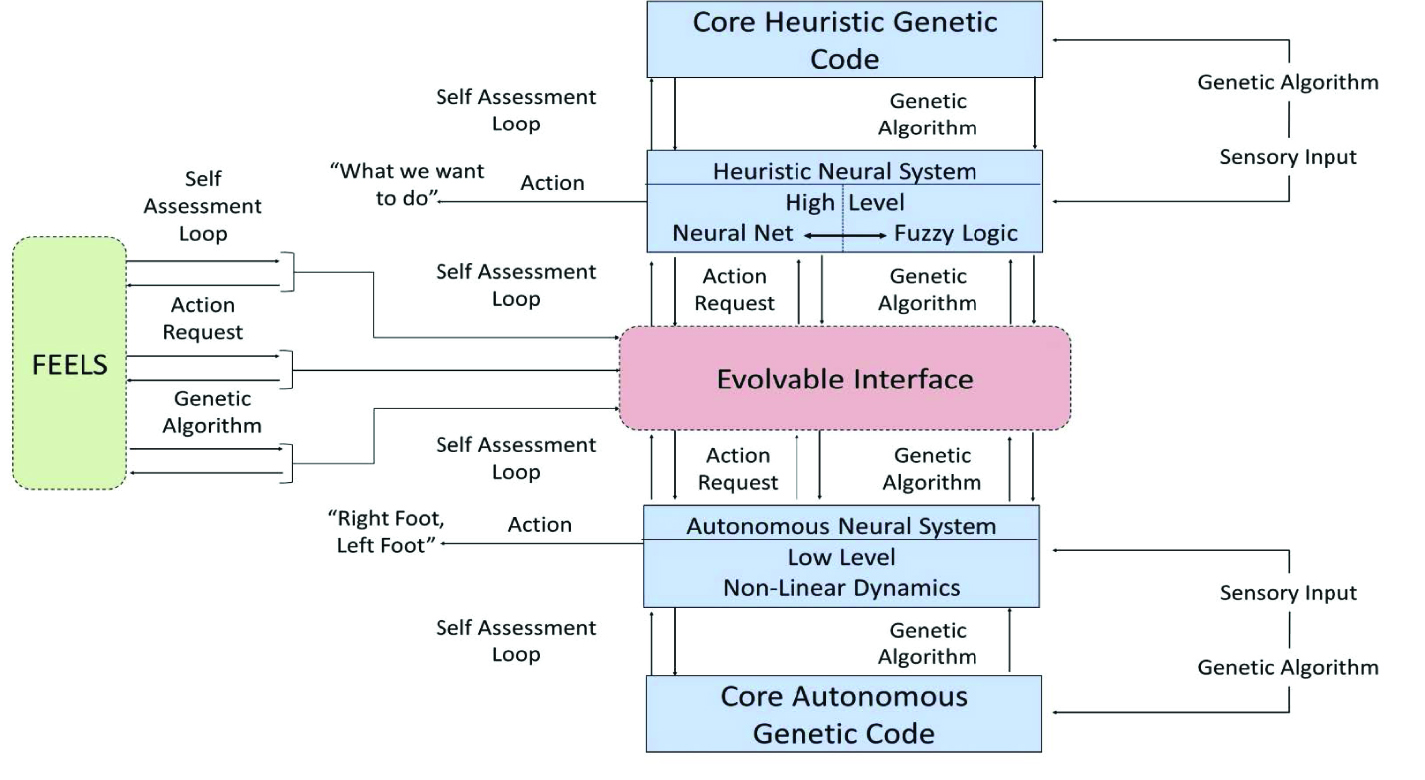
Benefits
- Performs with stability and predictability
- Enables reliability in autonomous systems
- Enables highly parallel processes, such as robotic swarms or large sensor networks
- Self-stabilizes: identifies and regulates unusual responses to inputs
- Self-corrects: corrects for errors such as a network node sending unusual or erroneous data
Applications
- Artificial intelligence research
- Sensor networks
- Autonomous spacecraft and robotics
- Autonomous network monitoring
Similar Results

Automata Learning in Generation of Scenario-Based Requirements in System Development
In addition, the higher the level of abstraction that developers can work from, as is afforded through the use of scenarios to describe system behavior, the less likely that a mismatch will occur between requirements and implementation and the more likely that the system can be validated. Working from a higher level of abstraction also provides that errors in the system are more easily caught, since developers can more easily see the big picture of the system.
This technology is a technique for fully tractable code generation from requirements, which has an application in other areas such as generation and verification of scripts and procedures, generation and verification of policies for autonomic systems, and may have future applications in the areas of security and software safety. The approach accepts requirements expressed as a set of scenarios and converts them to a process based description. The more complete the set of scenarios, the better the quality of the process based description that is generated. The proposed technology using automata learning to generate possible additional scenarios can be useful in completing the description of the requirements.

Closed Ecological System Network Data Collection, Analysis, Control, and Optimization System
The technology relates generally to controlled ecosystems, and more particularly, to a Controlled Closed-Ecosystem Development System (CCEDS) that can be used to develop designs for sustainable, small-scale reproductions of subsets of the Earths biosphere and the Orbiting Modular Artificial-Gravity Spacecraft (OMAGS). The technology encompassing a CCEDS includes one or more a Closed Ecological Systems (CESs), each having one or more Controlled Ecosystem Modules (CESMs). Each CESM can have a biome containing at least one organism, and equipment comprising one or more of sensors, actuators, or components that are associated with the biome. A controller operates the equipment to effect transfer of material among CESMs to optimize one or more CESM biomes with respect to their organism population health, resilience, variety, quantities, biomass, and sustainability. A CES is a community of organisms and their resources that persist in a sealed volume such that mass is not added or removed. The mass (food/air/water) required by the CES organisms is continually recycled from the mass (waste) produced by the organisms. Energy and information may be transferred to and from a CES. CES research promises to become a significant resource for the resolution of global ecology problems which have thus far been experimentally inaccessible and may very well prove an invaluable resource for predicting the probable ecological consequences of anthropogenic materials on regional ecosystems. In order to create CESs that are orders of magnitude smaller than the Earth that can function without the Earth, the desired gravity level and necessary radiation shielding must be provided by other means. Orbiting Modular Artificial-Gravity Spacecraft (OMAGS) is a fractional gravity spacecraft design for CES payloads and is depicted in Figures below. In tandem, the CCEDS and OMAGS systems can be used to foster gravitational ecosystem research for developing sustainable communities in space and on Earth.

Residual Mode Filters
Many control problems can benefit from the adaptive control algorithm described. This algorithm is well-suited to nonlinear applications that have unknown modeling parameters and poorly known operating conditions. Disturbance accommodation is a critical component of many systems. By using feedback control with disturbance accommodation, system performance and reliability can be increased considerably. Often the form of the disturbance is known, but the amplitude is unknown. For instance, a motor operating on a structure used for accurate pointing would cause a sinusoidal disturbance of a known frequency content. The algorithm described is able to accurately cancel these disturbances, without needing knowledge of their amplitude. In markets needing controllers, the efficiency, uptime, and lifespan of equipment can be dramatically increased due to the robustness of this technologys design.

Autonomic Autopoiesis
Highly distributed next-generation computer-based systems require self-managing environments that feature a range of autonomic computing techniques. This functionality is provided by collaborating agents, and includes an apoptotic (self-destruct) mechanism, autonomic quiescence (self-sleep), and others. The apoptotic feature is necessary to maintain system security and integrity when a component endangers the overall operation and viability of the entire system. However, the self-destruction of an agent/component may remove a key piece of functionality. The novel autopoietic functionality provides the capability to duplicate or substitute a new agent that provides the functionality of the self-destructed component.

System And Method for Managing Autonomous Entities through Apoptosis
In this method an autonomic entity manages a system through the generation of one or more stay alive signals by a hierarchical evolvable synthetic neural system. The generated signal is based on the current functioning status and operating state of the system and dictates whether the system will stay alive, initiate self-destruction, or initiate sleep mode. This method provides a solution to the long standing need for a synthetic autonomous entity capable of adapting itself to changing external environments and ceasing its own operation upon the occurrence of a predetermined condition deemed harmful.