Multivariate Monitoring for Human Operator and Machine Teaming
Sensors
Multivariate Monitoring for Human Operator and Machine Teaming (LAR-TOPS-301)
Instrumentation for biosignal, posture and behavioral gesture sensing for automation decision making
Overview
Researchers and expert operators may be familiar with the concept of trust in automation, but how would advance automation make decisions regarding control without establishing trust in the operator? Vehicles outfitted with sensors and systems that can operate with varying degrees of autonomy are being developed. Optimizing human machine interaction remains critical for maintaining and improving safety as vehicles become increasingly autonomous. Human status is highly variable and difficult to predict. Despite a recent history of consistent reliability, in the current moment the operator status may range from completely incapacitated to ready to take control as necessary or as preferred. The intelligent system itself needs to know what the human is doing now to make decisions in real time regarding role assignments, safe operation and critical functional task allocation.
The Technology
Inventors at NASA have developed a novel approach to optimizing human machine teaming. The technology enables the inclusion of the state of the human operator in system wide prognostics for increasingly autonomous vehicles. It also could inform the design of automation and intelligent systems for low proficiency and reduced crews. The system monitors and measures multiple variables in real time, the status of the human operator and communicates that information to an intelligent machine. Status could include behavior, skill, physical or medical status, or mental state. Once this information pathway is established, the predictability of pilot or operator status will be improved so the autonomous system can be said to develop trust in human operators much like humans develop trust in automation.
The system would utilize non-contact instrumentation for biosignal, posture and behavioral gesture sensing for automation decision making.
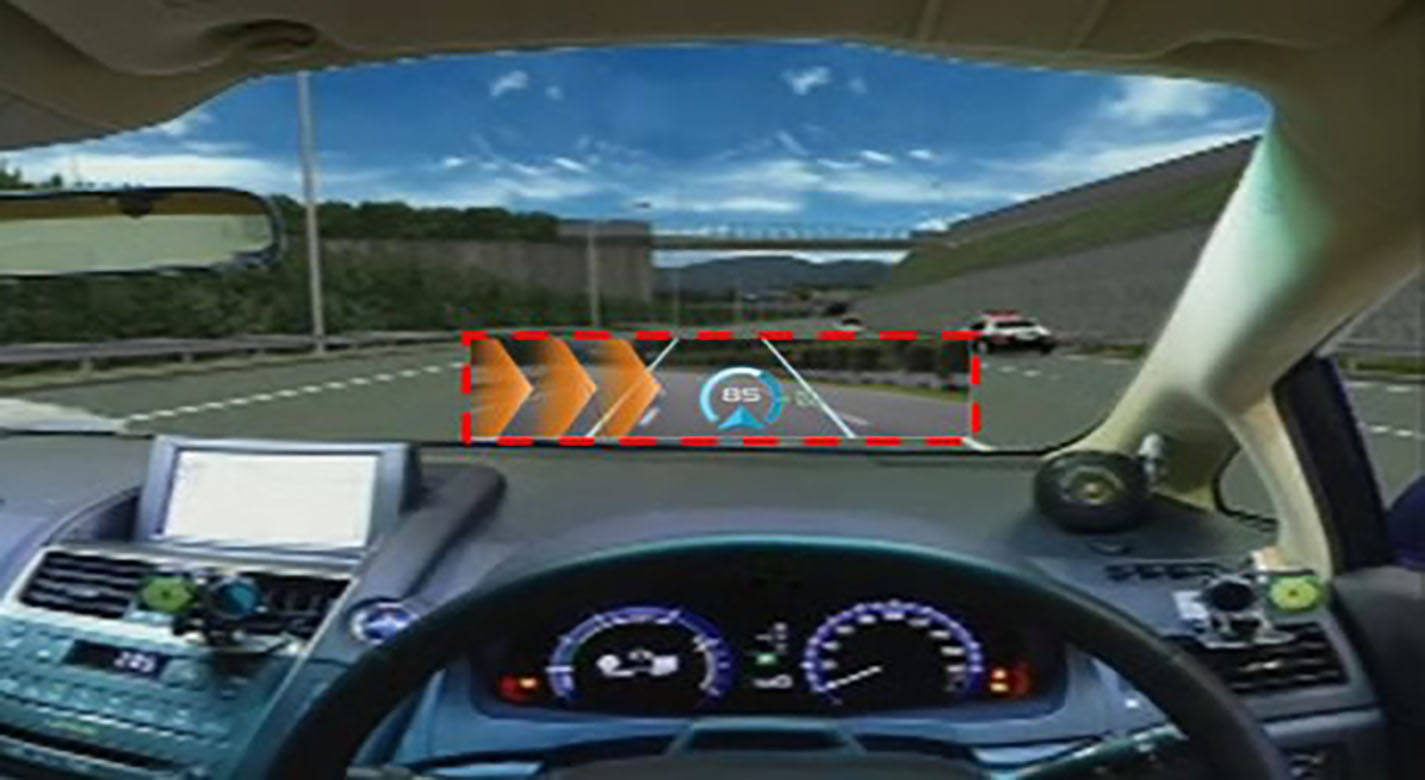
Benefits
- The system would utilize non-contact instrumentation for biosignal, posture and behavioral gesture sensing for automation decision making.
- Improved reliability of autonomous vehicles
- Can be designed to awaken a sleeping operator
- Informs the design of automation and intelligent systems for low-proficiency and reduced crews
- Improves the human-automation teaming and Includes the state of the human operator for increasingly autonomous vehicles
- Develop high-impact aviation autonomy applications
- Human-machine teaming in key applications
Applications
- Automotive – autonomous cars and other driverless vehicles
- Industrial automation
- Assessing commercial driver safety
- Monitoring of machine operator cognition
- Any other software where psychophysiological monitoring is useful
Technology Details
Sensors
LAR-TOPS-301
LAR-19051-1
LAR-19051-1-CON
LAR-19051-2-CON
LAR-19978-1-CIP
LAR-19978-1
Psychophysiological Sensing and State Classification for Attention Management in Commercial Aviation, Harrivel, A., Liles, C., Stephens, C., Ellis, K., Prinzel, L., Pope, A., AIAA Science and Technology Forum and Exposition, https://ntrs.nasa.gov/api/citations/20160007651/downloads/20160007651.pdf
Collaborative Communications Between a Human and a Resilient Safety Support System, Samani, Saeideh, Jessop, Richard, and Harrivel, Angela.,Institute of Electrical and Electronics Engineers, International Conference On Autonomous Systems Meeting, https://ntrs.nasa.gov/citations /20210010015
A ResNet Autoencoder Approach for Time Series Classification of Cognitive State, Terwilliger, Paul, Walker, Shannon, and Harrivel, Angela, MODSIM World 2020., https://modsimworld.org/papers/2020/MODSIM_2020_paper_53_.pdf
Collaborative Communications Between a Human and a Resilient Safety Support System, Samani, Saeideh, Jessop, Richard, and Harrivel, Angela.,Institute of Electrical and Electronics Engineers, International Conference On Autonomous Systems Meeting, https://ntrs.nasa.gov/citations /20210010015
A ResNet Autoencoder Approach for Time Series Classification of Cognitive State, Terwilliger, Paul, Walker, Shannon, and Harrivel, Angela, MODSIM World 2020., https://modsimworld.org/papers/2020/MODSIM_2020_paper_53_.pdf
Related Links:
|
Similar Results

Autonomic Autopoiesis
Highly distributed next-generation computer-based systems require self-managing environments that feature a range of autonomic computing techniques. This functionality is provided by collaborating agents, and includes an apoptotic (self-destruct) mechanism, autonomic quiescence (self-sleep), and others. The apoptotic feature is necessary to maintain system security and integrity when a component endangers the overall operation and viability of the entire system. However, the self-destruction of an agent/component may remove a key piece of functionality. The novel autopoietic functionality provides the capability to duplicate or substitute a new agent that provides the functionality of the self-destructed component.

Safe2Ditch Technology
Safe2Ditch is a crash management system that resides on a small processor onboard a small Unmanned Aerial Vehicle (UAV). The system's exclusive mission is emergency management to get the vehicle safely to the ground in the event of an unexpected critical flight issue. It uses the remaining control authority and battery life of the crippled vehicle in an optimal way to reach the safest ditch location possible. It performs this mission autonomously, without any assistance from a safety pilot or ground station. In the event of an imminent crash, Safe2Ditch uses its intelligent algorithms, knowledge of the local area, and knowledge of the disabled vehicle's remaining control authority to select and steer to a crash location that minimizes risk to people and property. As it approaches the site, it uses machine vision to inspect the selected site to ensure that it is clear as expected.

Near-Real Time Verification and Validation of Autonomous Flight Operations
NASA's Extensible Traffic Management (xTM) system allows for distributed management of the airspace where disparate entities collaborate to maintain a safe and accessible environment. This digital ecosystem relies on a common data generation and transfer framework enabled by well-defined data collection requirements, algorithms, protocols, and Application Programming Interfaces (APIs). The key components in this new paradigm are:
Data Standardization: Defines the list of data attributes/variables that are required to inform and safely perform the intended missions and operations.
Automated Real Time And/or Post-Flight Data Verification Process: Verifies system criteria, specifications, and data quality requirements using predefined, rule-based, or human-in-the-loop verification.
Autonomous Evolving Real Time And/or Post-Flight Data Validation Process: Validates data integrity, quantity, and quality for audit, oversight, and optimization.
The verification and validation process determines whether an operation’s performance, conformance, and compliance are within known variation. The technology can verify thousands of flight operations in near-real time or post flight in the span of a few minutes, depending on networking and computing capacity. In contrast, manual processing would have required hours, if not days, for a team of 2-3 experts to review an individual flight.

Algorithms for stabilizing intelligent networks
Some of the current challenges faced by research in artificial intelligence and autonomous control systems include providing self control, resilience, adaptability, and stability for intelligent systems, especially over a long period of time, in changing environments. The Evolvable Neural Software System (ENSS), Formulation for Emotion Embedding in Logic Systems (FEELS), Stability Algorithm for Neural Entities (SANE), and the Logic Expansion for Autonomously Reconfigurable Neural Systems (LEARNS) are foundations for tackling some of these challenges, by providing the basic algorithms evolvable systems could use to manage its own behavior.
These algorithms would allow networks to self regulate, noticing unusual behavior and the circumstances that may have caused that behavior, and then correcting to behave more predictably when similar circumstances are encountered. The process is similar to how psychology in organisms evolved iteratively, eventually finding and keeping better responses to given stimuli.

Airborne Machine Learning Estimates for Local Winds and Kinematics
The MAchine learning ESTimations for uRban Operations (MAESTRO) system is a novel approach that couples commodity sensors with advanced algorithms to provide real-time onboard local wind and kinematics estimations to a vehicle's guidance and navigation system. Sensors and computations are integrated in a novel way to predict local winds and promote safe operations in dynamic urban regions where Global Positioning System/Global Navigation Satellite System (GPS/GNSS) and other network communications may be unavailable or are difficult to obtain when surrounded by tall buildings due to multi-path reflections and signal diffusion. The system can be implemented onboard an Unmanned Aerial Systems (UAS) and once airborne, the system does not require communication with an external data source or the GPS/GNSS. Estimations of the local winds (speed and direction) are created using inputs from onboard sensors that scan the local building environment. This information can then be used by the onboard guidance and navigation system to determine safe and energy-efficient trajectories for operations in urban and suburban settings. The technology is robust to dynamic environments, input noise, missing data, and other uncertainties, and has been demonstrated successfully in lab experiments and computer simulations.