Interactive Diagnostic Modeling Evaluator
information technology and software
Interactive Diagnostic Modeling Evaluator (TOP2-245)
Patent Only, No Software Available For License.
Overview
NASA Ames has developed an interactive diagnostic modeling evaluator (i-DME) tool to aid in modeling for noise and lag in the data and debugging of system models when fault detection, isolation, and recovery results are incorrect. i-DME is designed to dramatically speed up the modeling debugging process. Often what hinders human-led model developments are, 1) the sheer size of playback files, 2) the modeling for noise and lag in the data, and, 3) debugging the fault/test relationships in the model. To alleviate these problems, i-DME can automatically playback very large data sets to find time points of interest where user-set performance criteria for detection and isolation are violated. i-DME modifies the diagnostic model through its abstract representation, diagnostic matrix (D-matrix). The types of modifications are procedures ranging from modifying 0s and 1s in the D-matrix adding/removing the rows/columns, or modifying test/wrapper logic used to determine test results. This software has the capacity to be applied to any complex system for navigation or generation of large amounts of complex data to identify, prioritize, and resolve errors in a self-correcting manner.
The Technology
The i-DME is a computer-user interactive procedure for repairing the system model through its abstract representation, diagnostic matrix (D-matrix) and then translating the changes back to the system model. The system model is a schematic representation of faults, tests, and their relationship in terms of nodes and arcs. D-matrix is derived from the system models propagation paths as the relationships between faults and tests. When the relation exists between fault and test, it is represented as 1 in the D-matrix. To repair the D-matrix and wrapper/test logic by playing back a sequence of nominal and failure scenarios (given), the user sets the performance criteria and accepts/declines the proposed repairs. During D-matrix repair, the interactive procedure includes conditions ranging from modifying 0s and 1s in the matrix, adding/removing the rows (failure sources) columns (tests), or modifying test/wrapper logic used to determine test results. The translation of changes to the system model is done via a process which maps each portion of the D-matrix model to the corresponding locations in the system model. Since the mapping back to the system model is non-unique, more than one candidate system model repair can be suggested. In addition to supporting the modification, it provides a trace for each modification such that a rational basis for each decision can be verified.
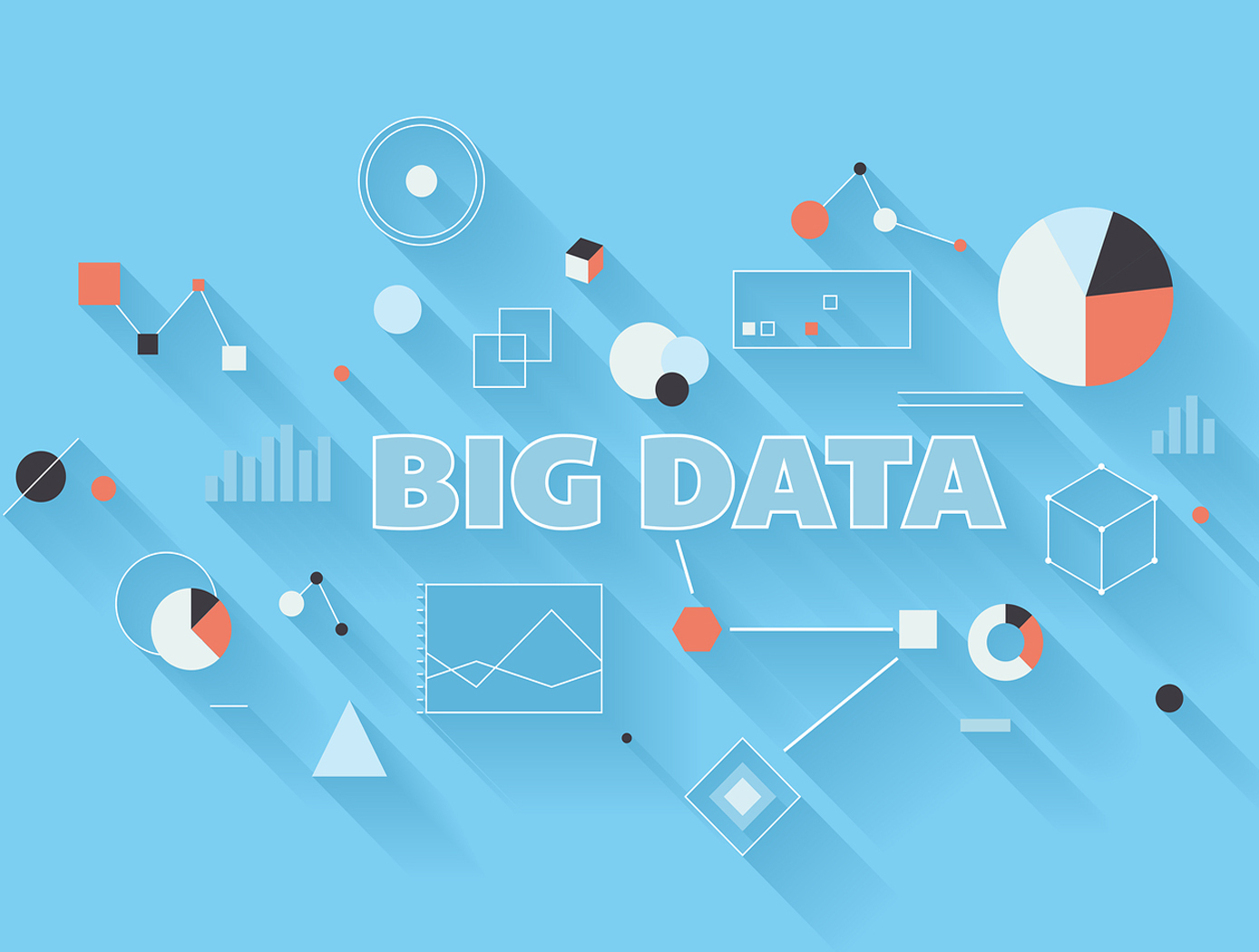
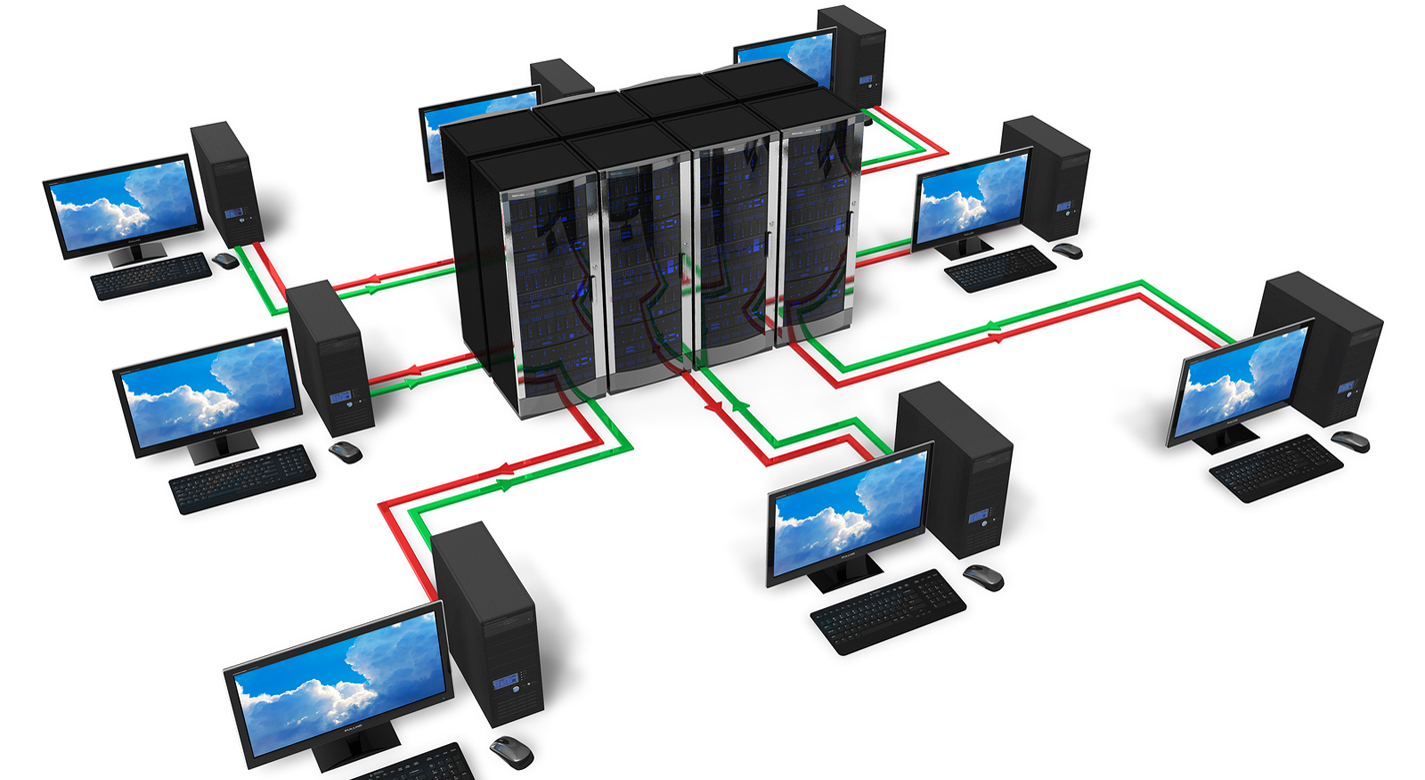
Benefits
- Cost effective modeling process
- Can be used in multiple areas
- Can automatically playback very large data sets
- Speeds up the modeling process
- Tracks all changes made over many iterations
Applications
- Industrial applications
- Consumer service applications
- Aerospace
- Chemical plant process monitoring
- Oil and gas plant operations
- Health monitoring systems
Similar Results

Enhancing Fault Isolation and Detection for Electric Powertrains of UAVs
The tool developed through this work merges information from the electric propulsion system design phase with diagnostic tools. Information from the failure mode and effect analysis (FMEA) from the system design phase is embedded within a Bayesian network (BN). Each node in the network can represent either a fault, failure mode, root cause or effect, and the causal relationships between different elements are described through the connecting edges.
This novel approach can help Fault Detection and Isolation (FDI), producing a framework capable of isolating the cause of sub-system level fault and degradation.
This system:
Identifies and quantifies the effects of the identified hazards, the severity and probability of their effects, their root cause, and the likelihood of each cause;
Uses a Bayesian framework for fault detection and isolation (FDI);
Based on the FDI output, estimates health of the faulty component and predicts the remaining useful life (RUL) by also performing uncertainty quantification (UQ);
Identifies potential electric powertrain hazards and performs a functional hazard analysis (FHA) for unmanned aerial vehicles (UAVs)/Urban Air Mobility (UAM) vehicles.
Despite being developed for and demonstrated with an application to an electric UAV, the methodology is generalized and can be implemented in other domains, ranging from manufacturing facilities to various autonomous vehicles.

Inductive Monitoring System
The Inductive Monitoring System (IMS) software provides a method of building an efficient system health monitoring software module by examining data covering the range of nominal system behavior in advance and using parameters derived from that data for the monitoring task. This software module also has the capability to adapt to the specific system being monitored by augmenting its monitoring database with initially suspect system parameter sets encountered during monitoring operations, which are later verified as nominal. While the system is offline, IMS learns nominal system behavior from archived system data sets collected from the monitored system or from accurate simulations of the system. This training phase automatically builds a model of nominal operations, and stores it in a knowledge base. The basic data structure of the IMS software algorithm is a vector of parameter values. Each vector is an ordered list of parameters collected from the monitored system by a data acquisition process. IMS then processes select data sets by formatting the data into a predefined vector format and building a knowledge base containing clusters of related value ranges for the vector parameters. In real time, IMS then monitors and displays information on the degree of deviation from nominal performance. The values collected from the monitored system for a given vector are compared to the clusters in the knowledge base. If all the values fall into or near the parameter ranges defined by one of these clusters, it is assumed to be nominal data since it matches previously observed nominal behavior. The IMS knowledge base can also be used for offline analysis of archived data.

Meta Monitoring System (MMS)
Meta Monitoring System (MMS) was developed as an add-on to NASA Ames patented Inductive Monitoring System (IMS), which estimates deviation from normal system operations. MMS helps to interpret deviation scores and determine whether anomalous behavior is transient or systemic. MMS has two phases: a model-building training phase, and a monitoring phase. MMS not only uses deviation scores from nominal data for training but can also make limited use of results from anomalous data. The invention builds two models: one of nominal deviation scores and one of anomalous deviation scores, each consisting of a probability distribution of deviation scores. After the models are built, incoming deviation scores from IMS (or a different monitoring system that produces deviation scores) are passed to the learned model, and probabilities of producing the observed deviation scores are calculated for both models. In this fashion, users of MMS can interpret deviation scores from the monitoring system more effectively, reducing false positives and negatives in anomaly detection.
Note: Patent license only; no developed software available for licensing

Dynamic Weather Routes Tool
Every 12 seconds, the Dynamic Weather Route (DWR) automation system computes and analyzes trajectories for en-route flights. DWR first identifies flights that could save 5 or more flying minutes (wind-corrected) by flying direct to a downstream return fix on their current flight plan. Eligible return fixes are limited so as not to take flights too far off their current route or interfere with arrival routings near the destination airport. Using the direct route as a reference route, DWR inserts up to two auxiliary waypoints as needed to find a minimum-delay reroute that avoids the weather and returns the flight to its planned route at the downstream fix. If a reroute is found that can save 5 minutes or more relative to the current flight plan, the flight is posted to a list displayed to the airline or FAA user. Auxiliary waypoints are defined using fix-radial-distance format, and a snap to nearby named fix option is available for todays voice-based communications. Users may also adjust the alert criteria, nominally set to 5 minutes, based on their workload and desired potential savings for their flights. A graphical user interface enables visualization of proposed routes on a traffic display and modification, if necessary, using point, click, and drag inputs. If needed, users can adjust the reroute parameters including the downstream return fix, any inserted auxiliary waypoints, and the maneuver start point. Reroute metrics, including flying time savings (or delay) relative to the current flight plan, proximity to current and forecast weather, downstream sector congestion, traffic conflicts, and conflicts with special use airspace are all updated dynamically as the user modifies a proposed route.

Residual Mode Filters
Many control problems can benefit from the adaptive control algorithm described. This algorithm is well-suited to nonlinear applications that have unknown modeling parameters and poorly known operating conditions. Disturbance accommodation is a critical component of many systems. By using feedback control with disturbance accommodation, system performance and reliability can be increased considerably. Often the form of the disturbance is known, but the amplitude is unknown. For instance, a motor operating on a structure used for accurate pointing would cause a sinusoidal disturbance of a known frequency content. The algorithm described is able to accurately cancel these disturbances, without needing knowledge of their amplitude. In markets needing controllers, the efficiency, uptime, and lifespan of equipment can be dramatically increased due to the robustness of this technologys design.