Inductive Monitoring System
information technology and software
Inductive Monitoring System (TOP2-175)
Automated monitoring techniques for complex systems
Overview
The Inductive Monitoring System (IMS) software utilizes techniques from the fields of model-based reasoning, machine learning, and data mining to build system monitoring knowledge bases from archived or simulated sensor data. Unlike some other machine learning techniques, IMS does not require examples of anomalous (failure) behavior. IMS automatically analyzes nominal system data to form general classes of expected system sensor values. This process enables the software to inductively learn and model nominal system
behavior. The generated data classes are then used to build a monitoring knowledge base. In real-time, IMS performs monitoring functions, determining and displaying the degree of deviation from nominal performance. IMS trend analyses can detect conditions that may indicate a failure or required system maintenance. The development of the IMS was motivated by the difficulty of producing detailed diagnostic models of some system components due to complexity or unavailability of design information. Previous and current IMS applications include the Hybrid Combustion Facility (HCF) advanced rocket fuel test facility, and the RASCAL UH-60 Blackhawk helicopter.
The Technology
The Inductive Monitoring System (IMS) software provides a method of building an efficient system health monitoring software module by examining data covering the range of nominal system behavior in advance and using parameters derived from that data for the monitoring task. This software module also has the capability to adapt to the specific system being monitored by augmenting its monitoring database with initially suspect system parameter sets encountered during monitoring operations, which are later verified as nominal. While the system is offline, IMS learns nominal system behavior from archived system data sets collected from the monitored system or from accurate simulations of the system. This training phase automatically builds a model of nominal operations, and stores it in a knowledge base. The basic data structure of the IMS software algorithm is a vector of parameter values. Each vector is an ordered list of parameters collected from the monitored system by a data acquisition process. IMS then processes select data sets by formatting the data into a predefined vector format and building a knowledge base containing clusters of related value ranges for the vector parameters. In real time, IMS then monitors and displays information on the degree of deviation from nominal performance. The values collected from the monitored system for a given vector are compared to the clusters in the knowledge base. If all the values fall into or near the parameter ranges defined by one of these clusters, it is assumed to be nominal data since it matches previously observed nominal behavior. The IMS knowledge base can also be used for offline analysis of archived data.
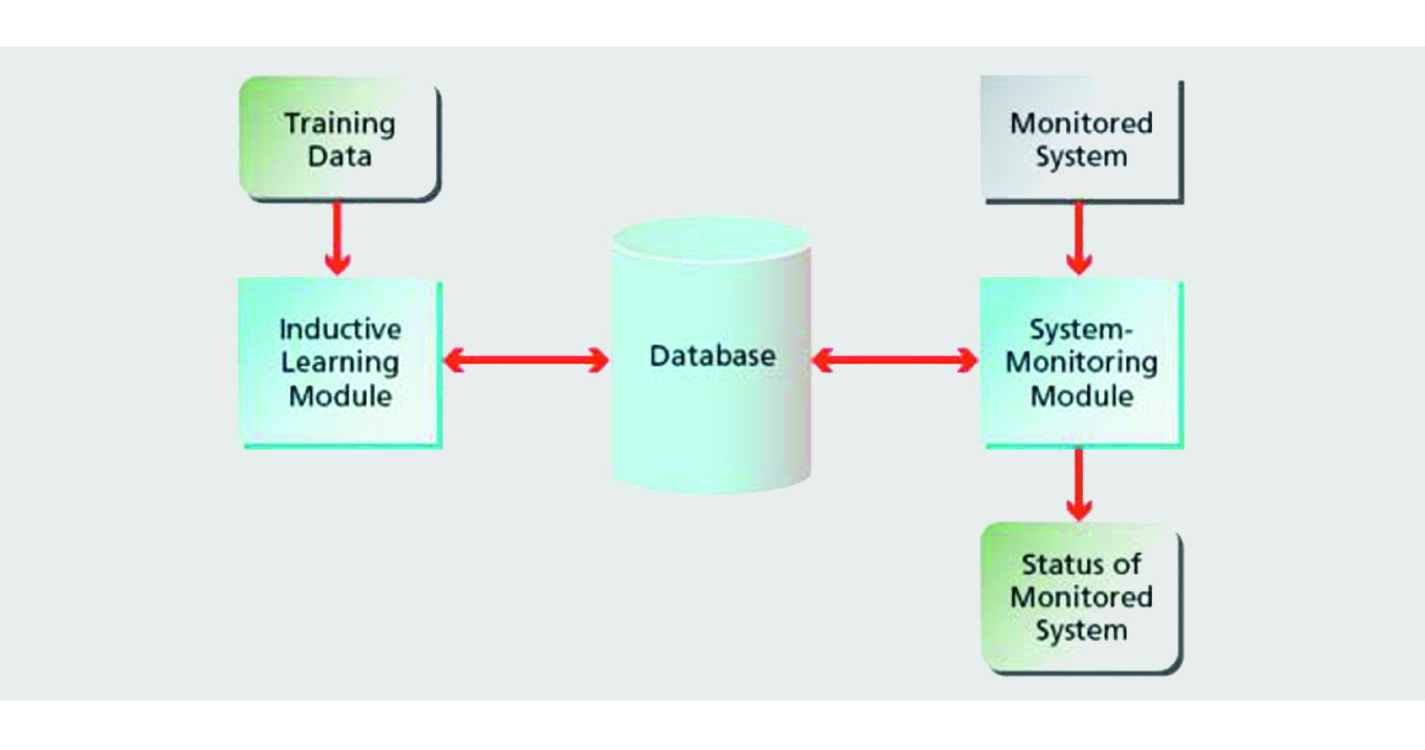
Benefits
- Decreases workload required to monitor system health and to respond to anomalous behavior
- Compact health information displays show degree of deviation from nominal performance
- Symbols encode information for quick interpretation
- Efficient and automatic - analyze full data sets
- Detected anomalies can be sent to diagnostic software
- Inductive learning
- Software re-use
Applications
- Aeronautics
- Space (on-board or mission control center)
- Surface transportation
- Medicine
- Research facilities and data
- Infrastructure
- Manufacturing/ process monitoring
- Military/security
Similar Results

Meta Monitoring System (MMS)
Meta Monitoring System (MMS) was developed as an add-on to NASA Ames patented Inductive Monitoring System (IMS), which estimates deviation from normal system operations. MMS helps to interpret deviation scores and determine whether anomalous behavior is transient or systemic. MMS has two phases: a model-building training phase, and a monitoring phase. MMS not only uses deviation scores from nominal data for training but can also make limited use of results from anomalous data. The invention builds two models: one of nominal deviation scores and one of anomalous deviation scores, each consisting of a probability distribution of deviation scores. After the models are built, incoming deviation scores from IMS (or a different monitoring system that produces deviation scores) are passed to the learned model, and probabilities of producing the observed deviation scores are calculated for both models. In this fashion, users of MMS can interpret deviation scores from the monitoring system more effectively, reducing false positives and negatives in anomaly detection.
Note: Patent license only; no developed software available for licensing

Mitigating Risk in Commercial Aviation Operations
NASA’s newly developed software leverages flight operations data (e.g., SWIM Terminal Data Distribution System (STDDS) information), and with it, can predict aviation related risks, such as unstable approaches of flights. To do this, the software inputs the complex, multi-source STDDS data, and outputs novel prediction and outcome information.
The software converts the relatively inaccessible SWIM data from its native format that is not data science friendly into a format easily readable by most programs. The converted, model friendly data are then input into machine learning algorithms to enable risk prediction capabilities. The backend software sends the machine learning algorithm results to the front end software to display the results in appropriate user interfaces. These user interfaces can be deployed on different platforms including mobile phones and desktop computers and efficiently update models based on changes in the data.
To allow for visualization, the software uses a commercially available mapping API. The data are visualized in several different ways, including a heat map layer that shows the risk score, with higher risk in areas of higher flight density, a polyline layer, which shows flight paths, and markers that can indicate a flight’s location in real time, among other things. The related patent is now available to license. Please note that NASA does not manufacturer products itself for commercial sale.

Interactive Diagnostic Modeling Evaluator
The i-DME is a computer-user interactive procedure for repairing the system model through its abstract representation, diagnostic matrix (D-matrix) and then translating the changes back to the system model. The system model is a schematic representation of faults, tests, and their relationship in terms of nodes and arcs. D-matrix is derived from the system models propagation paths as the relationships between faults and tests. When the relation exists between fault and test, it is represented as 1 in the D-matrix. To repair the D-matrix and wrapper/test logic by playing back a sequence of nominal and failure scenarios (given), the user sets the performance criteria and accepts/declines the proposed repairs. During D-matrix repair, the interactive procedure includes conditions ranging from modifying 0s and 1s in the matrix, adding/removing the rows (failure sources) columns (tests), or modifying test/wrapper logic used to determine test results. The translation of changes to the system model is done via a process which maps each portion of the D-matrix model to the corresponding locations in the system model. Since the mapping back to the system model is non-unique, more than one candidate system model repair can be suggested. In addition to supporting the modification, it provides a trace for each modification such that a rational basis for each decision can be verified.

Adaptive Algorithm and Software for Recognition of Ground-based, Airborne, Underground, and Underwater Low Frequency Events
Acoustical studies of atmospheric events like convective storms, shear-induced turbulence, acoustic gravity waves, microbursts, hurricanes, and clear air turbulence over the last forty-five years have established that these events are strong emitters of infrasound (sound at frequencies below 20 Hz). Over the years, NASA Langley has designed and developed a portable infrasonic detection system which can be used to make useful infrasound measurements at a location where it was not possible previously. The system comprises an electret condenser microphone, and a small, compact windscreen. The system has been modified to be used in the air, underground, as well as underwater (to determine man-made and precursor to tsunami). The system also features a data acquisition system that permits real-time detection, bearing, and signature of a low frequency source. However, to determine bearing of the received signals, the microphones are to be arranged as an equilateral triangle with a certain microphone spacing. The spacing depends upon location of the microphone array. For a ground-based array, the microphone spacing of 100 feet (30.48m) is desired to determine time delay for signals arriving at each microphone location. The microphone spacing depends upon speed of sound through the array medium. For underwater array, the spacing between microphones would be around 1500 feet. The data acquisition system provides data output in the infrasonic bandwidth which is then analyzed using an adaptive algorithm (least-mean-squares time-delay-estimation) using modern computational power to locate source by plotting source location hyperbolas on-line.
A smaller array size reduces the time resolution resulting in strong signal coherence. The innovation approach is to exploit modern signal processing methods, i.e. adaptive filtering, where computer is trained on-line to recognize features of the event to be detected. Modern computational capability permits the adaptive algorithm (least-mean-squares time-delay estimation or LMSTDE) which is vastly more powerful algorithm. This system has better resolution able to determine direction with arrived signals within five-degree accuracy.

System And Method for Managing Autonomous Entities through Apoptosis
In this method an autonomic entity manages a system through the generation of one or more stay alive signals by a hierarchical evolvable synthetic neural system. The generated signal is based on the current functioning status and operating state of the system and dictates whether the system will stay alive, initiate self-destruction, or initiate sleep mode. This method provides a solution to the long standing need for a synthetic autonomous entity capable of adapting itself to changing external environments and ceasing its own operation upon the occurrence of a predetermined condition deemed harmful.