Search
Optics
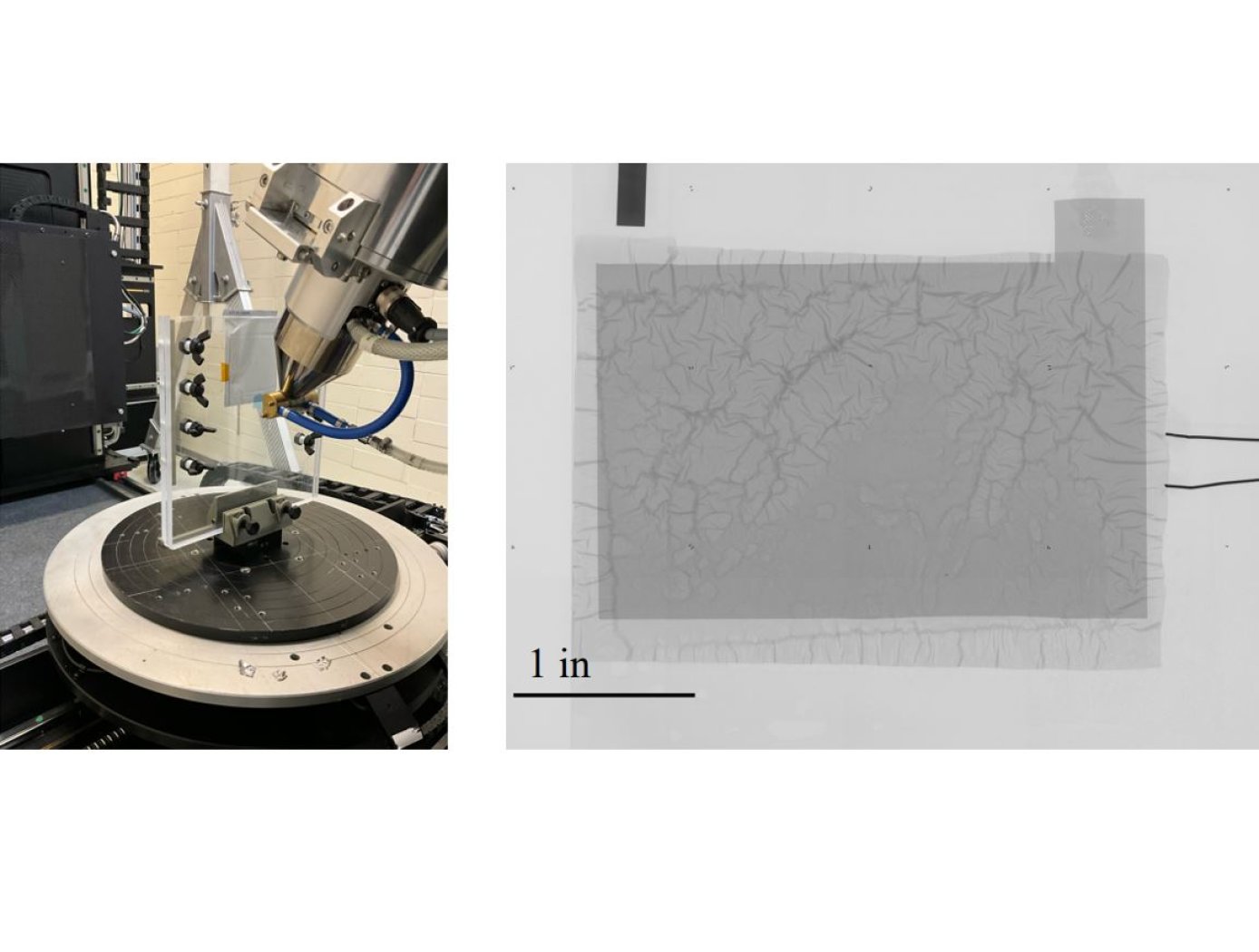
Fingerprinting for Rapid Battery Inspection
The technology utilizes photopolymer droplets (invisible to the digital radiograph) with embedded radiopaque fragments to create randomized fingerprints on battery samples. The droplets are deposited using a jig (see figure on right) that precisely positions samples. Then, at different points during battery R&D testing or use, digital radiography imaging with micron-level resolution can be performed.
The high-resolution imaging required to detect dendrite formation requires images to be collected in multiple “tiles” as shown below. The randomized fingerprints uniquely identify relative positioning of these tiles, allowing rapid assembly of composite high-resolution images from multiple tiles.
This same composite creation process can be used for images taken at a series of points in time during testing, and background subtraction can be applied to efficiently compare how the battery is changing over successive charge/discharge cycles to identify dendrite formation. This inspection technique is proven effective for thin-film pouch cell prototypes at NASA, and it works well at the lowest available x-ray energy level (limiting impact on the samples). The Fingerprinting for Rapid Battery Inspection technology is available for patent licensing.
information technology and software
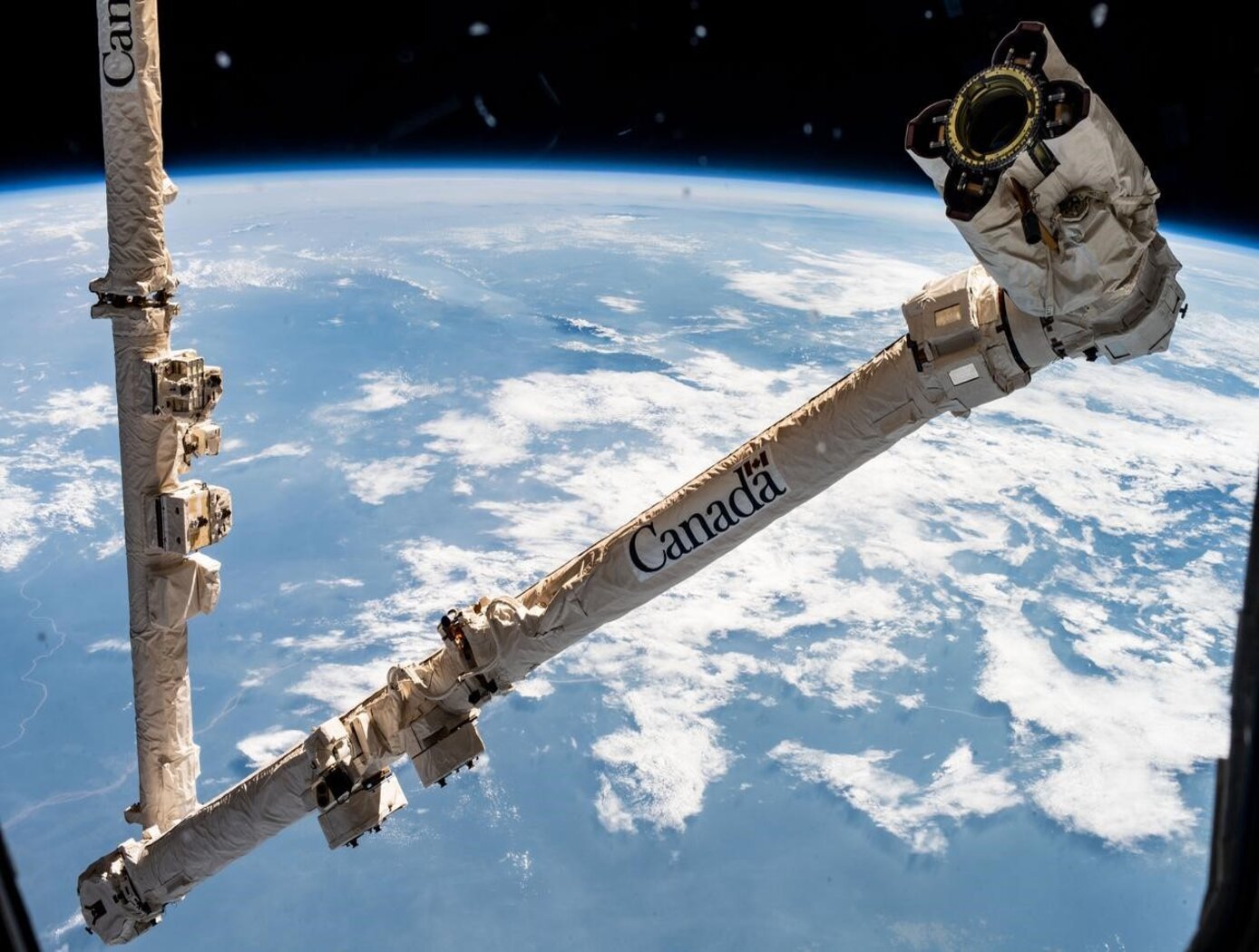
Computer Vision Lends Precision to Robotic Grappling
The goal of this computer vision software is to take the guesswork out of grapple operations aboard the ISS by providing a robotic arm operator with real-time pose estimation of the grapple fixtures relative to the robotic arms end effectors. To solve this Perspective-n-Point challenge, the software uses computer vision algorithms to determine alignment solutions between the position of the camera eyepoint with the position of the end effector as the borescope camera sensors are typically located several centimeters from their respective end effector grasping mechanisms.
The software includes a machine learning component that uses a trained regional Convolutional Neural Network (r-CNN) to provide the capability to analyze a live camera feed to determine ISS fixture targets a robotic arm operator can interact with on orbit. This feature is intended to increase the grappling operational range of ISSs main robotic arm from a previous maximum of 0.5 meters for certain target types, to greater than 1.5 meters, while significantly reducing computation times for grasping operations.
Industrial automation and robotics applications that rely on computer vision solutions may find value in this softwares capabilities. A wide range of emerging terrestrial robotic applications, outside of controlled environments, may also find value in the dynamic object recognition and state determination capabilities of this technology as successfully demonstrated by NASA on-orbit.
This computer vision software is at a technology readiness level (TRL) 6, (system/sub-system model or prototype demonstration in an operational environment.), and the software is now available to license. Please note that NASA does not manufacture products itself for commercial sale.
Manufacturing

X-Ray Crack Detectability
NASAs software technology uses an Image Quality Indicator (IQI)-based model that can predict whether cracks of a certain size can be detected, as well as a model that can provide appropriate conditions to optimize x-ray crack detection setup. Because this modeling software can predict minimum crack sizes that can be detected by a particular X-ray radiography testing setup, users can test various setups until the desired crack detection capabilities are achieved (predicted) by the modeling system.
These flaw size parameter models use a set of measured inputs, including thickness sensitivity, detector modulation transfer function, detector signal response function, and other setup geometry parameters, to predict the minimum crack sizes detectable by the testing setup and X-ray angle limits for detecting such flaws.
Current X-ray methods provide adequate control for detection of volumetric flaws but do not provide a high probability of detection (POD), and crack detection sensitivity cannot be verified for reliable detection. This results in reduced confidence in terms of crack detection. Given that these cracks, if undetected, can cause catastrophic failure in various systems (e.g., pressure vessels, etc.), verifying that X-ray radiography systems used for NDE can detect such cracks is of the utmost importance in many applications.